It’s no secret that data governance has a bad rap with business leaders. For some people, it evokes images of bureaucracy, data locked up in secret vaults and endless time spent on meetings and updating spreadsheets only to see no result of their hard work.
Despite the very known importance of making the data readily available, relevant and high quality, many organisations still fail to grasp the value of it and have a solid data governance programme in place in 2020.
Even enterprises that have made efforts towards creating a governance framework haven’t seen any significant returns from it. The main problem McKinsey defines is that the most data governance programmes today are ineffective, which originates in that the C-suite executives don’t recognise the value potential of data governance. As a consequence, it is demoted to a set of policies and guidance that support a function executed by IT and not followed organisation-wide, which renders the data-powered initiative equally ineffective, McKinsey identifies.
The consequences are immense, as organisations not only miss out on data-driven opportunities, but they also waste precious resources on data processing and cleanup which consumes the majority of the analytics and data science team’s time. This also impacts the scalability of data projects and declined productivity of teams. Also, companies that haven’t invested in a proper data governance strategy are putting their organisations at high regulatory risks.
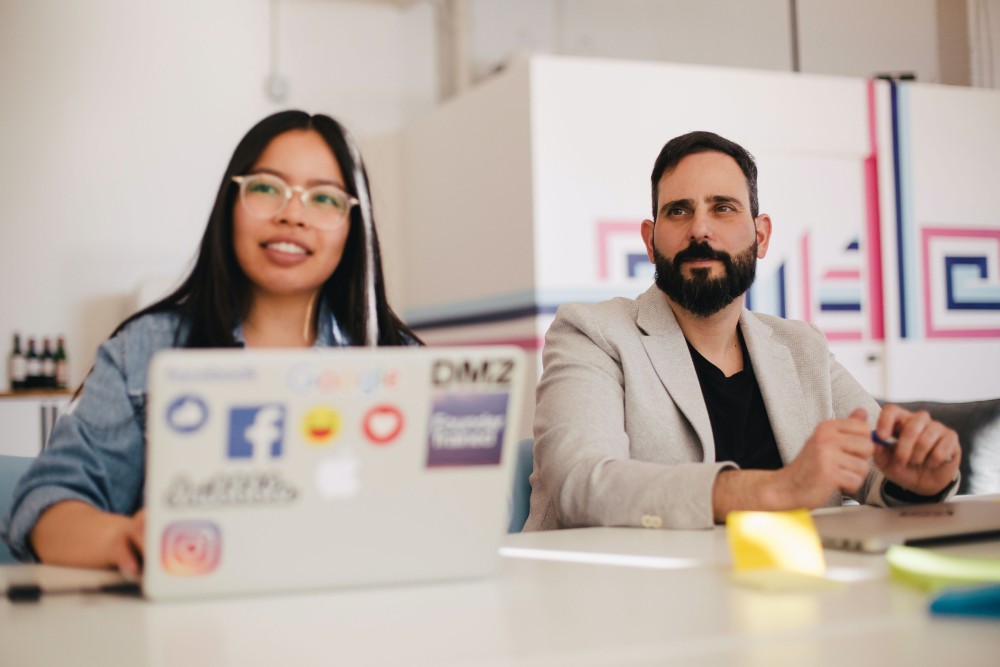
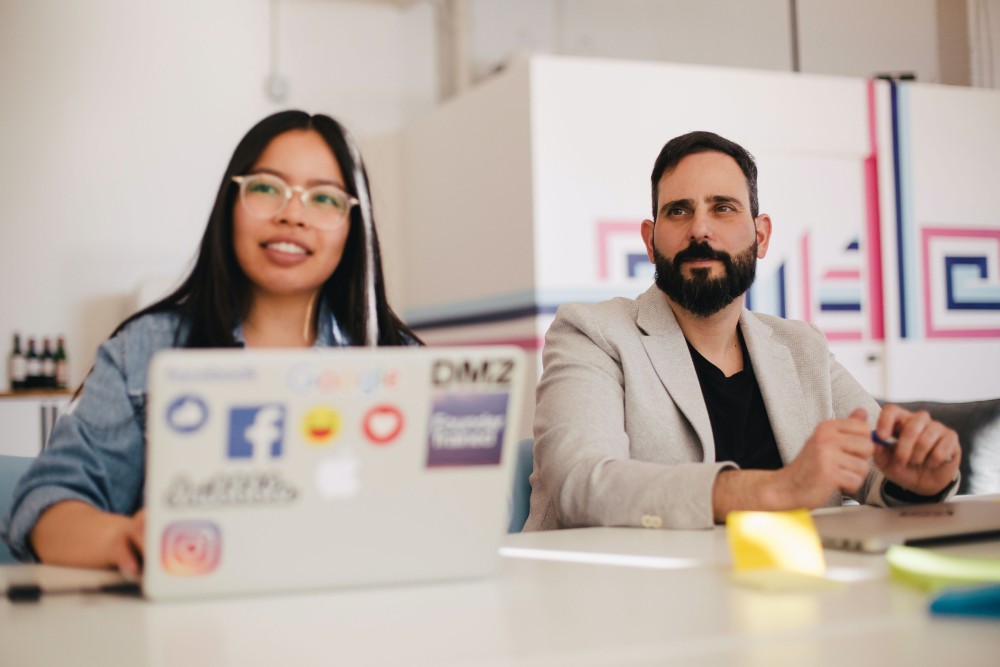
Why data governance practices haven’t worked
The failures of governance projects and organisations’ reluctance to implement it stem from misguided past experiences and broken practices. It’s not uncommon to see data lake projects being started without the “boring, old” data governance, because it’s just “a bunch of slides full of theory” that no one follows and is just a lot of work that will only slow down the exciting new data project.
However, as data lake projects progress, they slowly start transforming into what looks more like data swamps stirred by the poor data quality and unreliable insights that don’t show any value for the business. An initial solution is to reach out to a data catalogue that would help identify what data is in there, what it means, who owns it, who is using it and where it came from.
Although data catalogues may be an easy fix, and it’s indeed a sign of moving the needle, it doesn’t replace the reinforcement of having bespoke data governance in place. So far, data governance has staggered because the technology wasn’t mature enough or companies couldn’t stimulate people to follow procedures and processes because of negative associations with the term governance.
In another case, companies rushed to solve data governance problems by using technology. And sure, technology solutions such as data lakes and data-governance platforms do help, but they are not the magical concoction to fix all governance problems. Tools, platforms and solutions cannot be discussed unless there is a clear strategy on the table. Implementation data governance is hard work, and there’s no way around it. It means creating new roles and responsibilities, transformed cultures, and streamlined workflows.
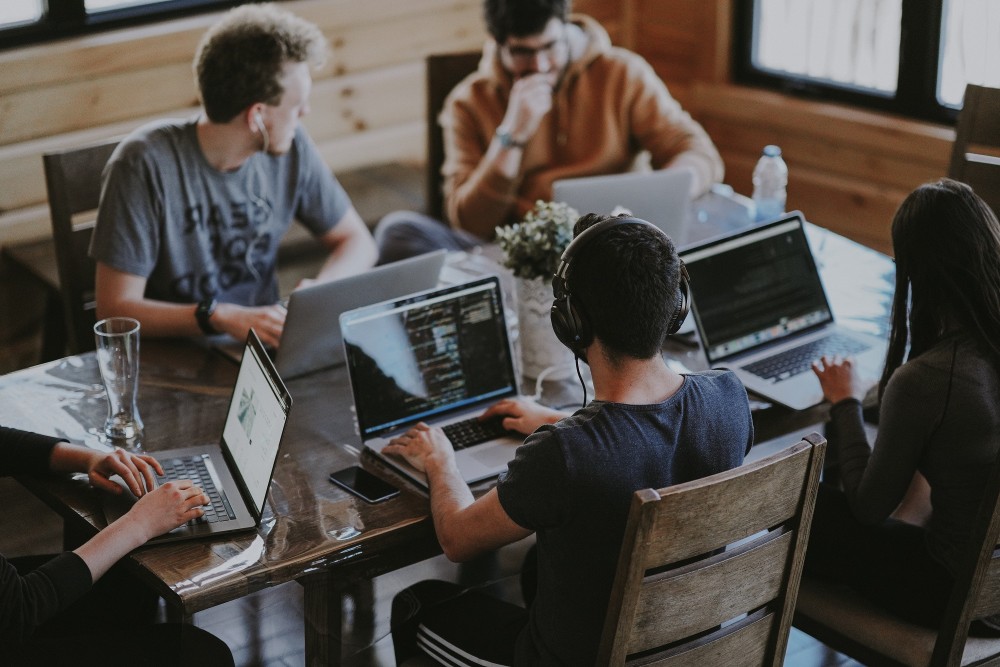
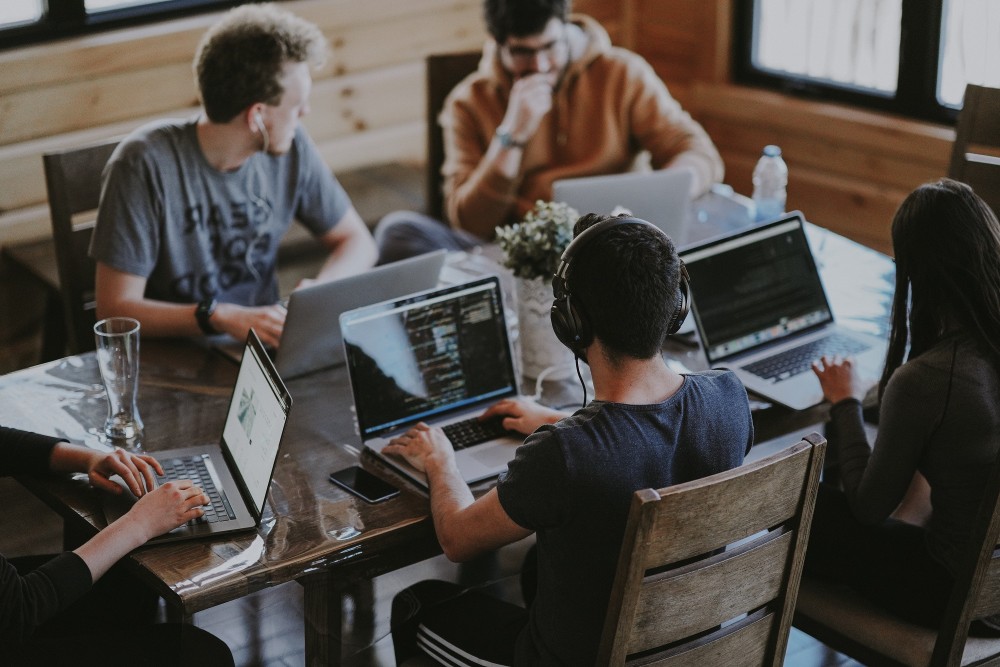
In this context, data experts indicate that a new transformation emerges boosted by the recent advances in data lake tooling which promises to reimagine the way we govern data (i.e. the culture, structures, and processes in place to achieve the risk mitigation and cost take out from governance). Ryan Gross described the transformation resulting in “data governance [that] will look a lot more like DevOps, with data stewards, scientists, and engineers working closely together to codify the governance policies throughout the data analytics lifecycle”.
In fact, the fruits of the governance transformation have been present in the field in the DataOps methodology which builds upon the application of DevOps practices to modern data management and integration. When DataOps is applied to data projects, it helps reduce the cycle time of data analytics in close alignment with business objectives.
Combining the practices of DevOps, agile development and lean manufacturing, DataOps empowers continuous design of data solutions, continuous data operations and continuous governance responsible for establishing an Information Governance framework, a methodology, and standards for enterprise information management.
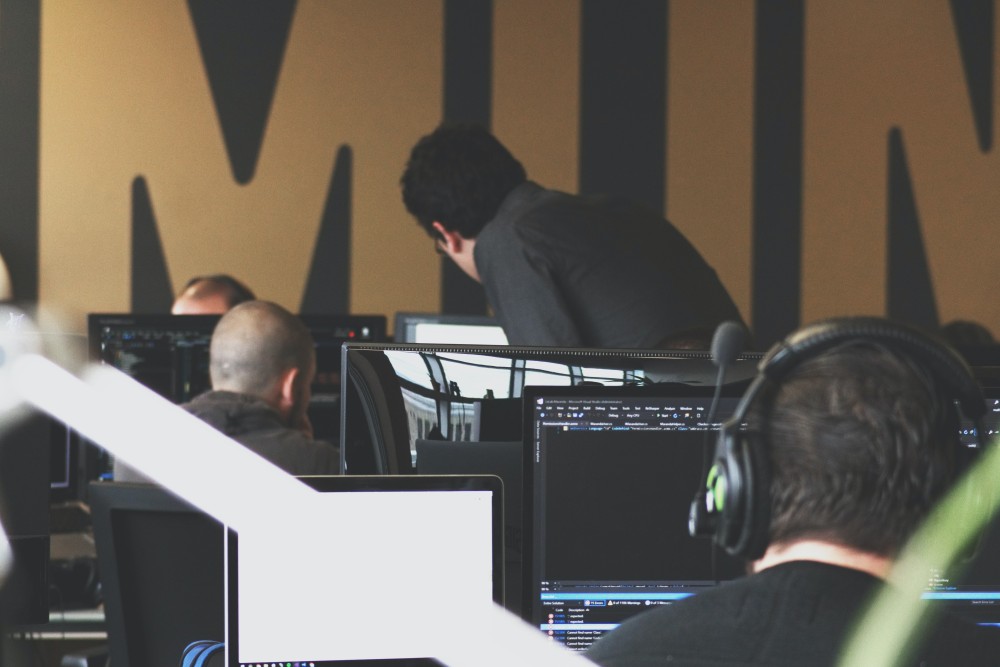
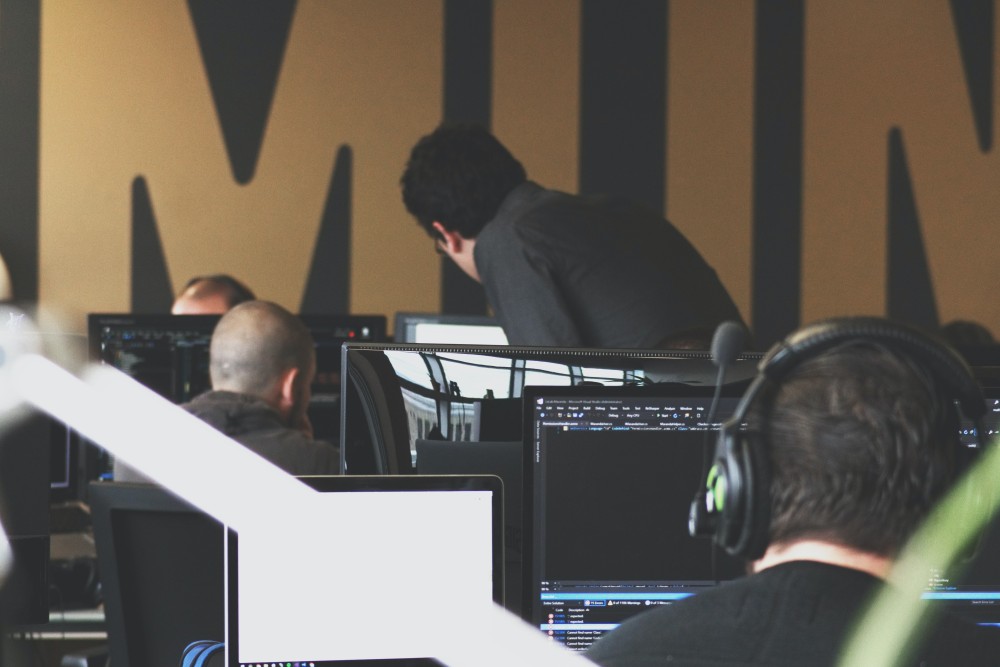
How DataOps introduces successful data governance
In a paper at the beginning of 2020, 451 Research reported that the DataOps momentum is soaring among companies, with an almost unprecedented 100% of respondents said they are currently planning (28%) or actively pursuing (72%) initiatives to deliver more agile and automated data management. The survey revealed that the main focus areas for enterprises’ DataOps-related spending were Analytics and Self-Service Data Access (40%), Data Virtualization (37%), Data Preparation (32%), Data Lake/Fabric (On-Premises or Public Cloud) (25%) and Metadata Management and Data Governance (23%), among others.
In regards to DataOps in a governance context, the paper outlines that one of the primary aims for DataOps for governance is improving the ability to respond to regulatory requirements, besides establishing the governance rules that can enable the acceleration of analytics initiatives.
According to 451 Research report, companies that have a more mature approach to data governance see an acceleration of analytics initiatives. They also note a positive approach to data governance among enterprises, treating it as providing the guardrails to move faster with self-service and agile analytics.
This widespread adoption trend corresponds to greater maturity in relation to data governance and DataOps.
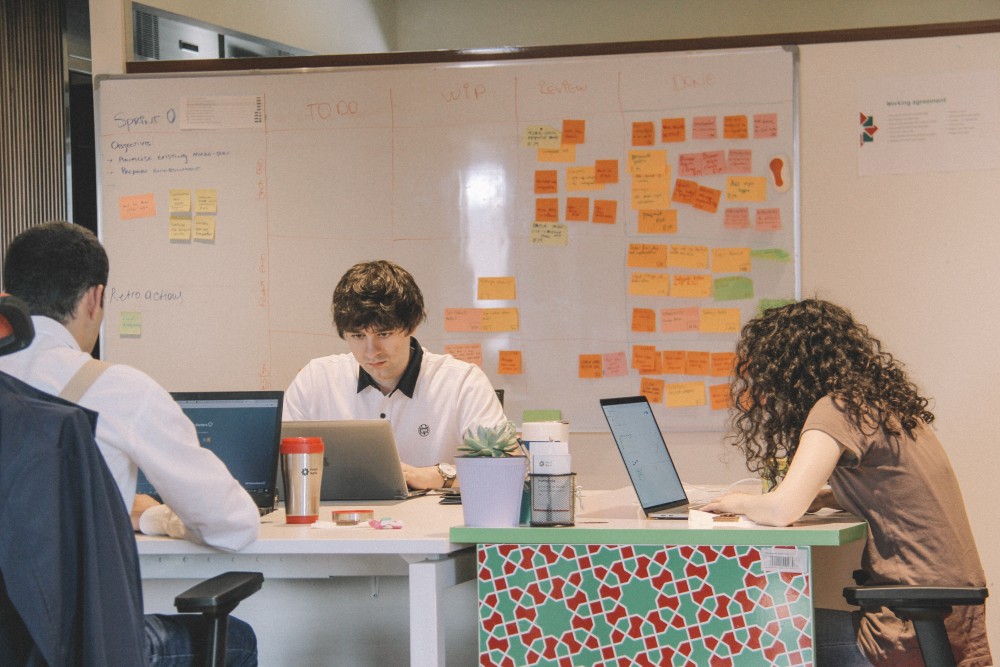
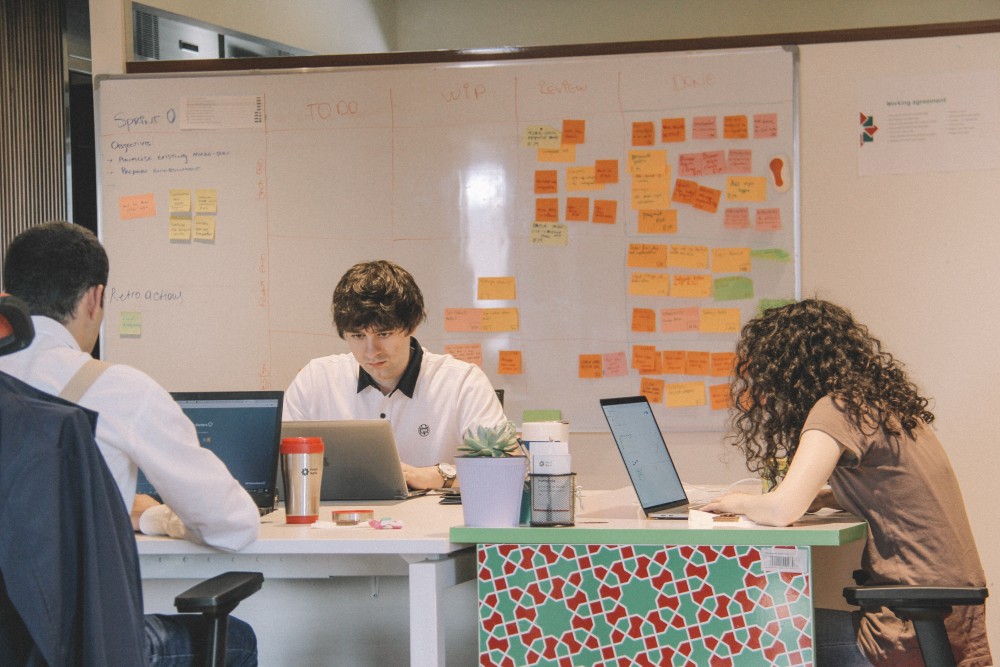
How DataOps delivers value: IBM case study
When examining real case studies where DataOps results in more efficient data governance, we turn to IBM, where the term DataOps was introduced. Namely, Lenny Liebmann, then working as a Contributing Editor at InformationWeek, for the first time mentioned the term DataOps in a blog post on the IBM Big Data & Analytics Hub titled “3 reasons why DataOps is essential for big data success” in 2014.
Fast forward 5 years to last year’s Data 2020 Summit, Julie Lockner, Director, Data and AI Portfolio Operations, Customer Experience and Offering Management at IBM, talked about how DataOps delivers value to data governance and integration initiatives through several success stories.
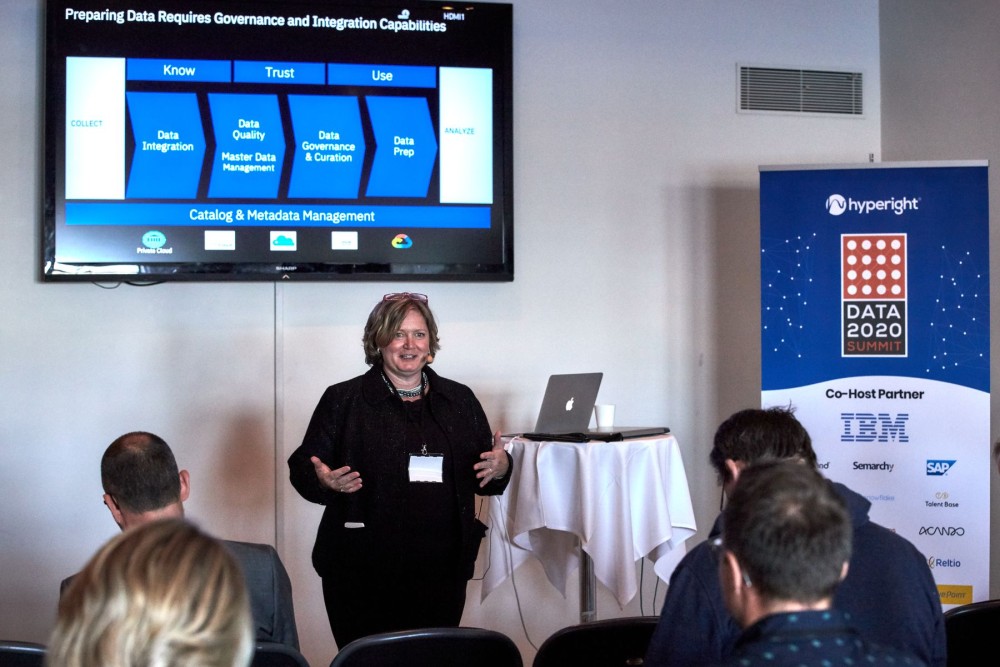
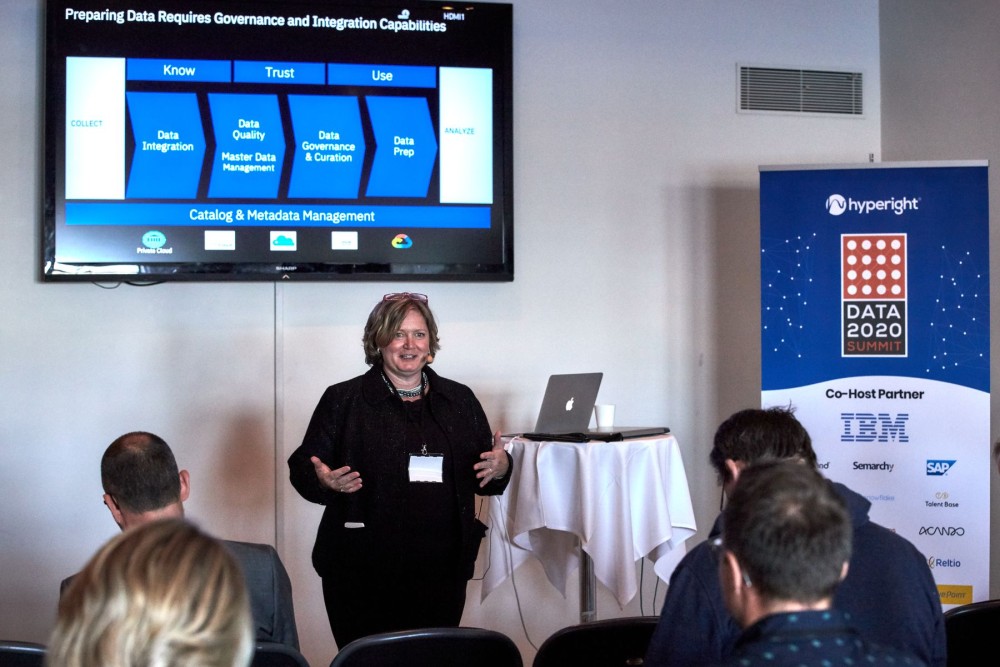
Julie Lockner referred to Gartner’s definition of DataOps describing it as a collaborative data management practice focused on improving communication, integration and automation of data flow between data managers and data consumers across an organisation. But, she also added that apart from data flows, it also implies the context in which data flows. For example, in a case when an artefact changes, people know why it changed in the first place, who is responsible for it, how to fix all down steam reports, who replaces the responsible person if they are absent.
On top of everything, companies are in constant conflict to make data available for the users and to meet regulatory compliance requirements, and security and privacy policies, states Julie. Just as DevOps proved successful in providing a solution for IT operations and application security, applying the same methodology to data operations brings the already proven efficiency.
In fact, if we present DevOps, DataOps and AIOps (the emerging practice of applying artificial intelligence (AI) to enhance IT operations), data governance underpins all operations for continuous innovation and delivery, whether with applications, data or AI and analytics.
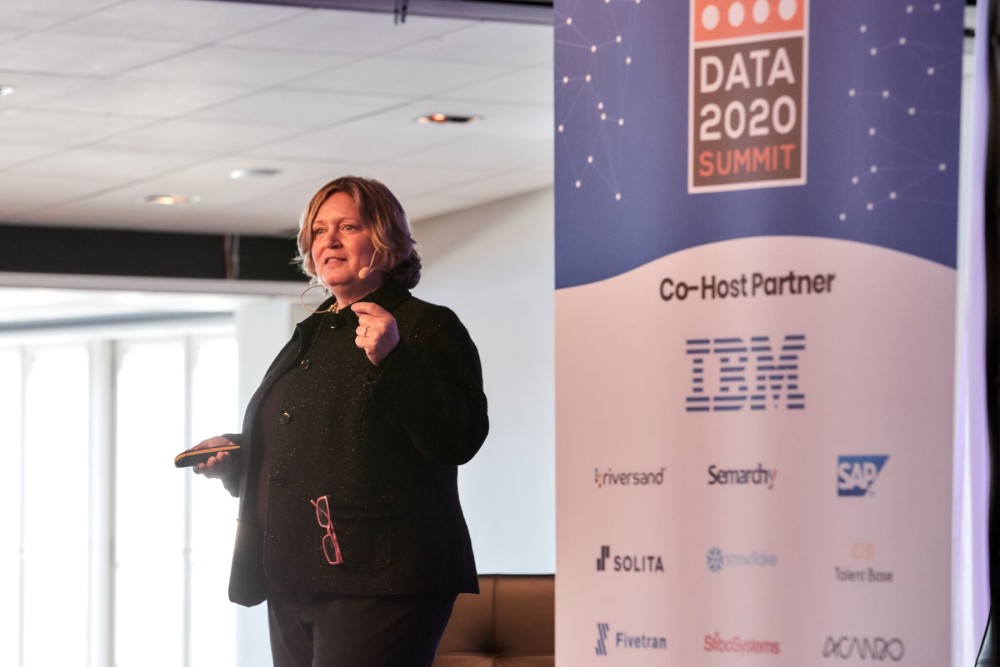
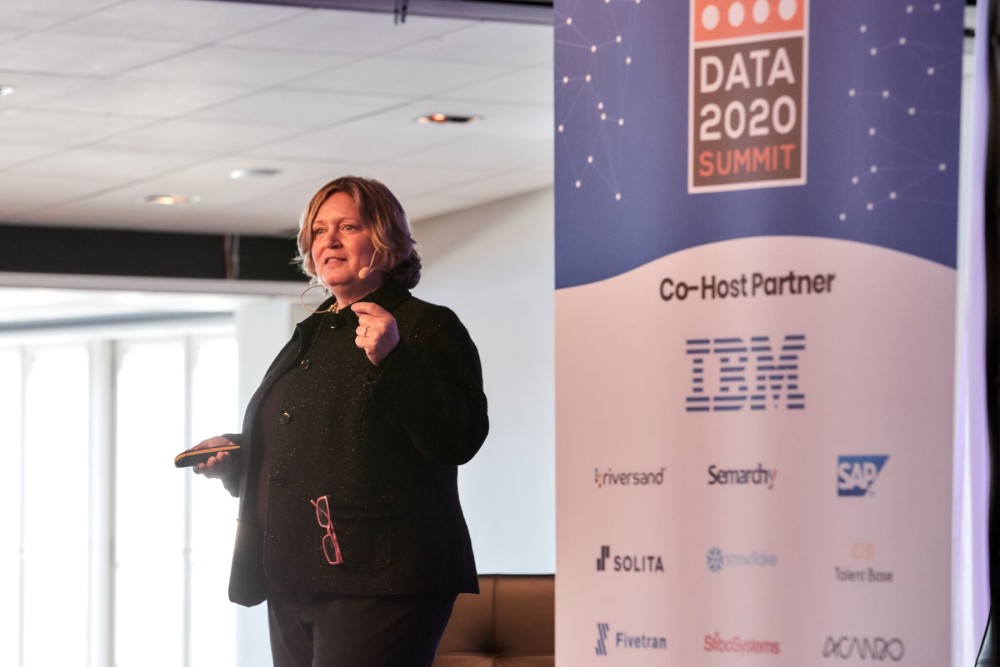
Data governance is naturally integrated into all of them, points out Julie. It is not a set of intimidating rules that have to be enforced in operations. Or in Julie Lockner’s words, DataOps in action is when data managers know what data they have, they know where it came from, they can trust it and make it available for data users.
In the end, a successful DataOps put into practice should consistently deliver high-quality data sets fast by streamlining data pipeline processes.
Add comment