“How can we make AI our main business so that it’s not just a Proof of Concept or a pilot but it’s actually our earning machine? On the one hand, we have new AI research happening – new models, new algorithms, new techniques – but then on the other hand we really need entire business model innovation, new ways of doing business, new ways of creating, delivering and capturing value.”
The wise words from Girish Agarwal, ex-Director of the AI Lab at Husqvarna and current Chief Information and Digital Officer at Piab, during our conversation on Altair’s ‘Future Says’. Away from his industry work, Girish is also a PhD fellow at KTH-Stockholm researching how AI is transforming customer value perception and disrupting existing business models. This business model transformation, which he has rolled out at Husqvarna and is beginning at Piab, formed the basis of our exciting conversation.
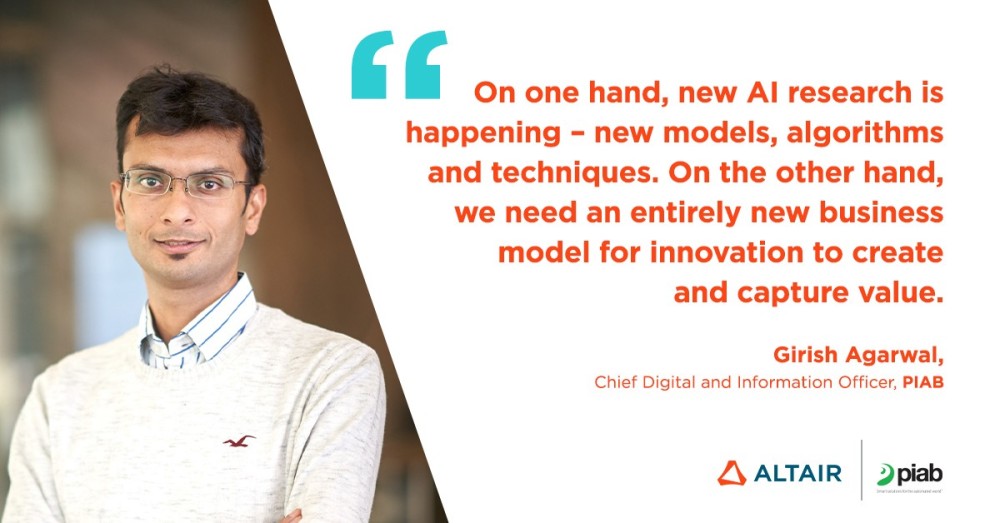
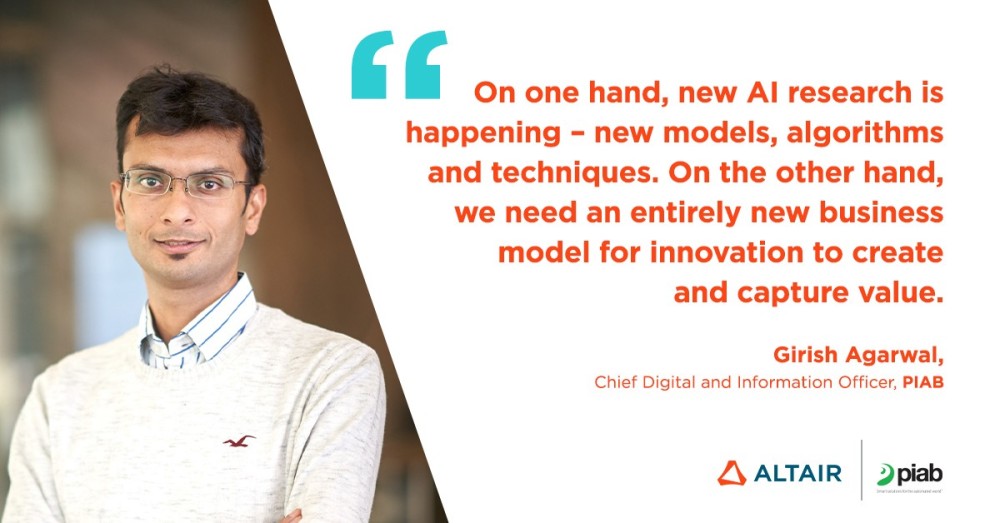
Despite the global impact of Covid-19, AI investments have been largely unchanged since the start of the pandemic. With all this investment, perhaps you would have expected that all major corporations are already reaping big rewards from AI, but that is simply not the case. In fact, a recent survey from McKinsey stated that only 22% of companies using AI have reported a sizeable bottom-line impact. Similarly, according to research from Bain, up to 87% of executives are said to be unsatisfied with their company’s current approach to AI. Clearly, something has to change, and it is time for organisations to take a step back and re-define their structure and business models to fully exploit AI’s potential value.
Defining a new Organisational Structure
No two companies will adopt AI in the same way as every organisation has its own aspirations, strengths, and weaknesses to consider. However, whatever approach you adopt, it is important to ensure cross-organisational communication, collaboration, and a centralised coordination of AI initiatives. At Husqvarna, they have divided this structure into an AI Research Lab and an AI Service Department:
“AI research is doing quick iteration projects, six to eight weeks. After two, three, four iterations, when we can make something out of it, that is when it goes into the AI service delivery model, wherein we help business to incorporate this.”
A Centre of Excellence (CoE), like Husqvarna’s, will help bring a sustained focus to AI, but you should always remain laser-focused on enabling AI throughout the organisation, not just in small, central teams. Accordingly, the role of the CoE should become smaller as the whole company begins applying data in their daily processes.
It is not just about the structure of this initiative but also the culture change that needs to present itself alongside. In its 2019 annual survey, Gartner asked CDO’s about their biggest obstacles to gaining value from analytics. The result was culture. As examples, you must shift workers away from traditional mindsets, like relying on top-down decision making, and replace static, annual project planning cycles with a more dynamic approach.
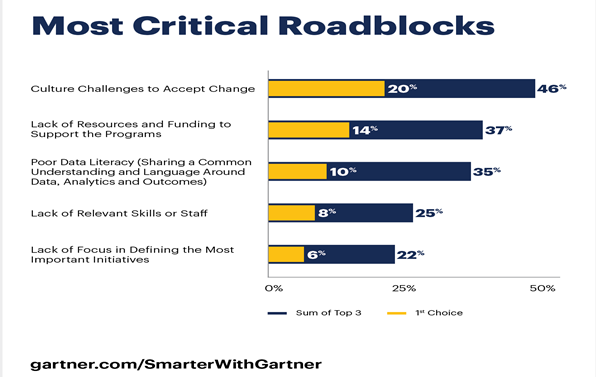
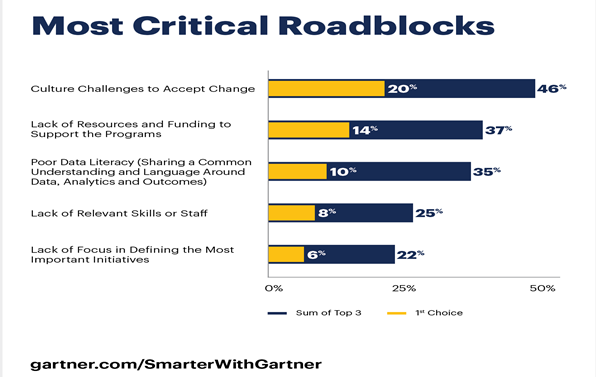
Ultimately, it is about reorganising your business around innovation and addressing the customer desires today and into the future. To help manage this, here are a few key strategies to keep in mind.
- Start Small, Start Early
Don’t worry if you are still in the early stages of AI maturity. You are not alone. Research shows that the majority of companies (80-85%) are still in the initial Proof of Concept (PoC) stage. Girish’s main recommendation was to immediately get started on this journey:
“The right time to start is now – If you don’t have data it’s not a showstopper, if you don’t have a digital strategy it’s not a showstopper, if you don’t have a digital platform it’s not a showstopper, and if you don’t have the right competence it’s not a showstopper. Simply get started, have an open mindset, and keep learning as you go along.”
Begin by identifying opportunities for AI within your organisation. A good place to start is the typical manual, time-consuming, and error-prone tasks. If you are still not sure where to start, look to your industry competitors for inspiration. Next, structure a short pilot program around one of these use cases, just like Girish and Husqvarna:
“None of the projects that we run in the lab are more than seven, maximum eight weeks. And when we say a project, we do not make PowerPoints, we make end to end prototypes. In those eight weeks, you have to identify the business use case, the problem, and the data that can give us insights into this. Something tangible needs to come out really quickly.”
Remember that pursuing initiatives that are excessively difficult or time-consuming can sabotage current and future projects. Oftentimes, companies opt for either the ‘low hanging fruit’ or the ‘all in’ strategy but my advice is to always develop a portfolio of initiatives with different time horizons. Get the best of both worlds – early wins and longer-term, strategic transformations.
2. Scale Fast
Of course, using AI for one-off experiments is not exploiting its full value so if the results are positive, you are now ready to move towards production and expansion to other products and service lines. In Gartner’s 2020 Hype cycle for AI, they identify 2 megatrends within the AI landscape: democratisation and industrialisation. Girish’s advice was:
“Don’t get stopped or stalled by PoC’s. Take them to the last step, take them to your business, take them to your customers. Don’t be satisfied with running pilots, try to industrialise them.”
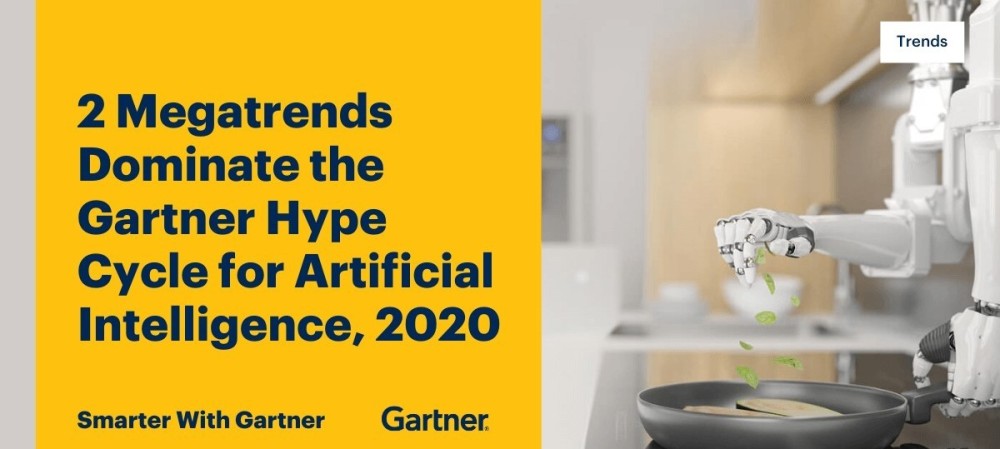
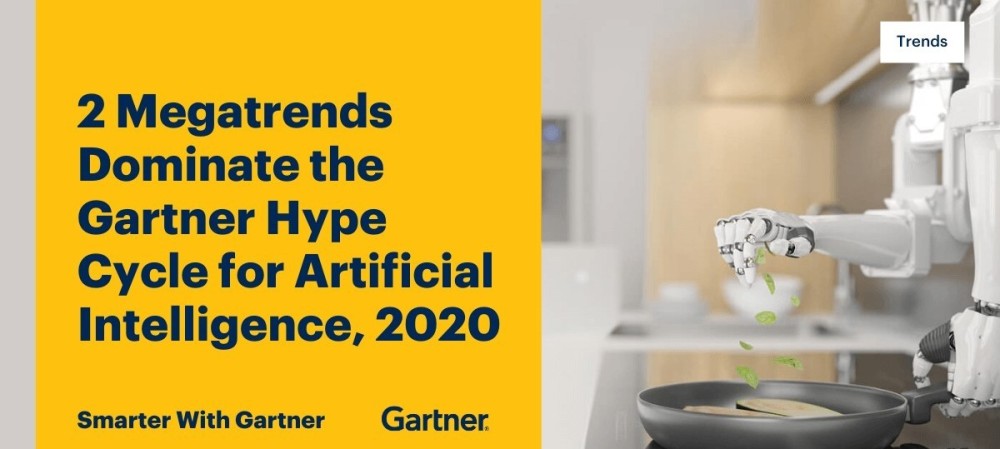
In truth, it is deceivingly easy to build an AI PoC but implementing and scaling it within operational processes, throughout an enterprise, is much more complex. At Husqvarna, only one in five projects make it to their AI Service Delivery. Organisations must shed the mindset that an idea needs to be fully considered before deploying. Try not get stuck in the minutiae. A trial-and-error mentality will re-frame mistakes as sources of discoveries, thereby reducing the fear of failure. Consider starting a ‘lessons learned’ conversation with stakeholders to review what went wrong and what can be done better in future initiatives. This will speed up development, enabling small data teams to create MVP’s (Minimum Viable Products) in weeks rather than months.
A 2019 study conducted by Accenture found that 86% of executives believed they would not achieve their growth objectives unless they can scale AI projects. What’s worse, three quarters of these C-Suite executives believe that if they don’t not move beyond the PoC, they risk going out of business by 2025. Despite these sobering statistics, too often companies are falling into the trap of being satisfied running basic pilot projects. You must snap out of this process. Remember to ‘Nail it, then scale it.’
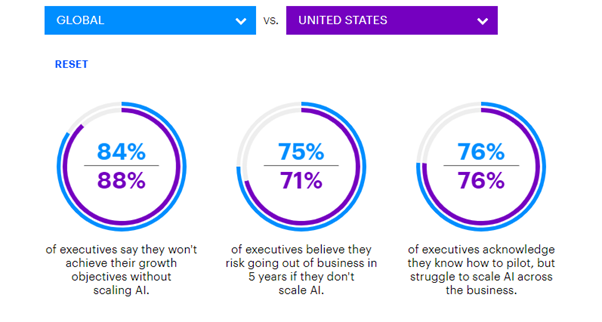
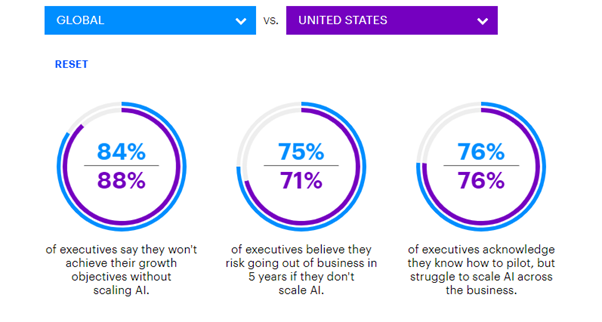
3. Democratise Access
Furthermore, it is a common misconception that one unicorn developer can do all of this work. In reality, you need many people with complementary skills who are already enabled in your organisation. Think of AI as a team sport. Training needs to take place and organisations need to be redesigned to accommodate a new data-centric way of working. Girish painted the picture at Husqvarna:
“We have 22,000 dealers / mechanical engineers. They know how to configure a two stroke four stroke piston. If I ask them to configure my AI model, that is not going to work. On the other hand, we are a bunch of technologists who are good at data-driven technologies, but we don’t know our customers and their problems. It’s our business teams who have been working with our customers for 20 – 25 years. We have to anchor ourselves with them. We build this competence through democratisation and citizen data scientists. If we have one ML expert, we try to have five to eight people from the business who can anchor together with them.”
Change your recruiting techniques now to create the workforce you will need in five years. Seek people who are enthusiastic to champion AI across the organisation. Develop a flexible learning and development program which maps career paths and include regular employee assessment points to maintain momentum. Another thing you should keep an eye on, according to Girish, is the gig economy:
“I think this is also going to play a major role in our ambitions with resourcing in this area – how to integrate ourselves with the gig economy, wherein all of a sudden, you have access to 55 million developers in the world who are ready to work with you.”
According to a report from Microsoft released last year, UK companies are not doing enough to prepare their workforce for the increased use of AI. Statistics show that UK leadership focus on the technology itself before the skills of the people using it. Companies desperately need to understand that its people are the ones that will generate future success and that they need to be brought more directly into the development fold.
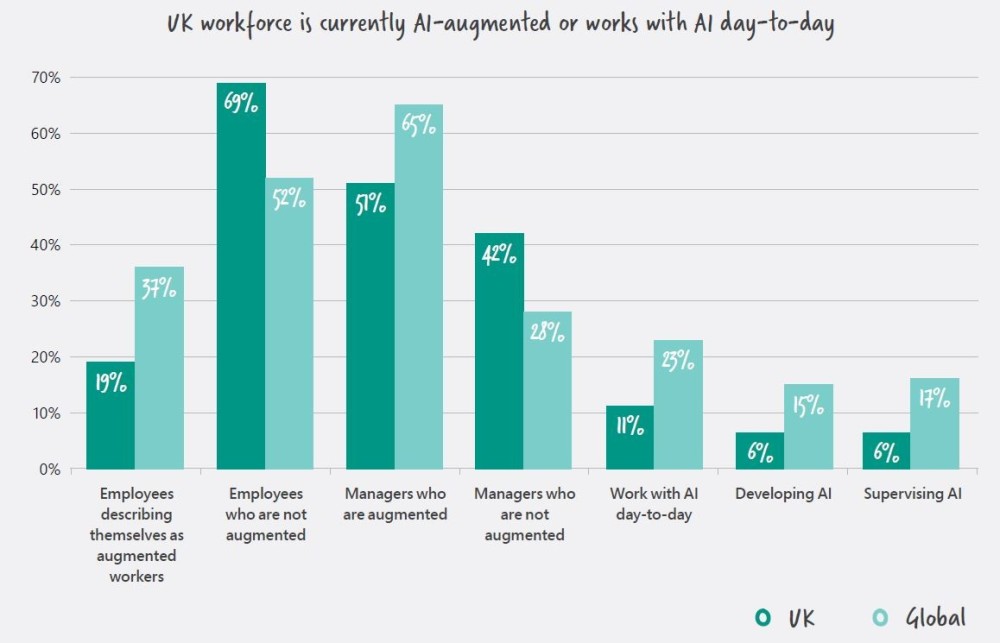
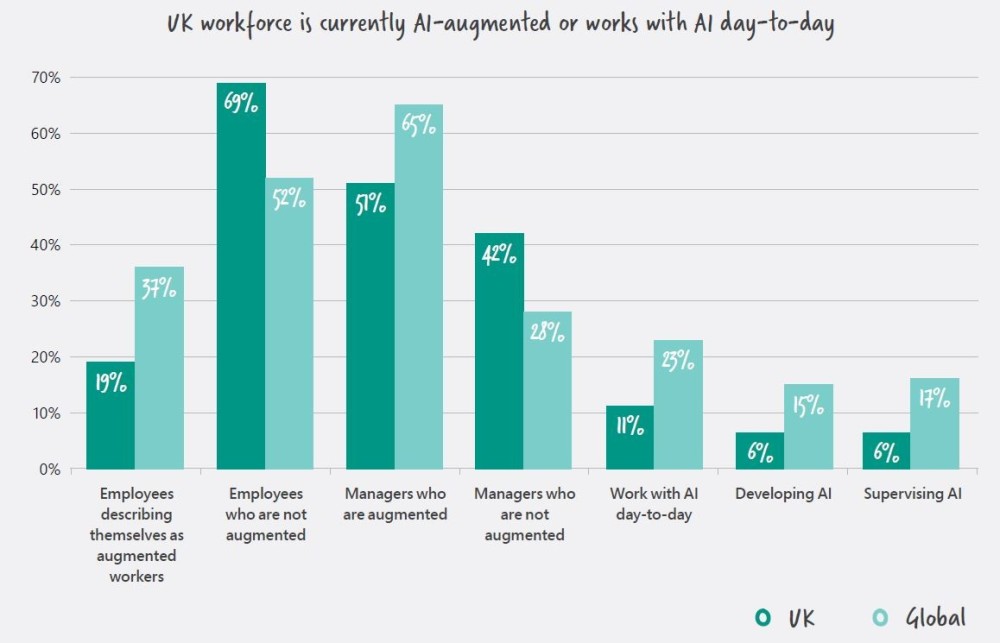
4. Leadership
For AI to scale, we will also need effective leadership. A common problem I see is senior management believing that AI is a magic bullet whereas, in reality, you need to put a lot of effort into acquiring and cleansing data before you even think about modelling and analysis. It is not simply plug-and-play. With a fresh emphasis on data democratisation, leaders must actively encourage new ways of working. AI requires experimentation, and early iterations often do not work out as expected. When that happens, leaders must highlight the lessons learned and continue the momentum. There are few better people in the European data science community to talk about leadership than Girish. He said:
“Leadership will make or break this acceleration. To lead such a transformation, you really need leaders who are challenging the status quo, who have the mindset and approach for failing, who can stand up in front of management. For me, the definition of failure has changed. As far as we are learning, we are not failing.”
One of the easiest steps for facilitating a practical understanding of AI is for leadership to simply demystify it by explaining how employees can use the technology to amplify their day-to-day efficiency and effectiveness. Do not think about ‘AI use cases’, think about business use cases that could incorporate AI. Without strategic alignment, you will likely see a sporadic set of isolated initiatives rather than a holistic approach to utilising AI to fundamentally disrupt businesses. Despite this, McKinsey’s State of AI report, still states that only 30 percent of organisations are aligning their AI strategy with the corporate strategy. As Girish stressed:
“Technology remains technology – the interesting thing is how to make value for customers using technology.”
Put simply, a lot of organisations are still spending vast sums of money wholly in the name of AI. Leaders must strive to demonstrate economic value from AI while shaping the organisational culture to facilitate this data-driven transformation.
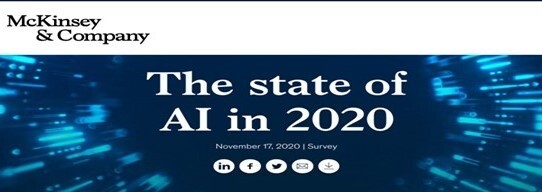
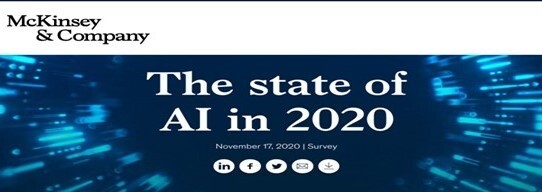
5. Identify long-term partners
Vendor partnerships will accelerate innovation and speed to market, but AI transformations are long-term projects involving engineering, training, and entirely overhauling business processes, so you must choose partners wisely. Here are some aspects to consider:
- Industry Experience: Cut through the hype and identify companies with customer stories or relevant experience.
- Ease-of-Use: Code-free and code-friendly technology can empower data scientists, encourage citizen data scientists, and excite senior executives. Open-source technology can be volatile, lack transparency and be overly complex to implement.
- Functionality Depth: Introducing AI into processes can require additional effort at any stage from data preparation to data mining, machine learning, model deployment, monitoring, and management. Your AI platform should scale to different use cases – simple data preparation in back-office finance, reporting dashboards at high-level management and complex advanced analytics within the lines of business.
- Flexibility: You will likely need to host your platform in the cloud, on-premise or a combination of both. Equally important to flexibility in deployment is scalability to the constantly increasing volume, velocity, veracity, and variety of data.
- Support: The partnership should not conclude at the point of purchase. A ‘Mutual Success Plan’ should be documented with the vendor to ensure a healthy, long-term engagement.
- Cost Transparency: Make sure you clearly map all requirements at inception including any expected future costs, such as model updates or adding data sources.
Of course, the required functionality needs to be as flexible and iterative as the technologies that drives them. Girish compared data science to web development in 1991 when we would lock “technical writers, content developers, web developers, web architects and business guys in a room for six weeks and out came 25 pages of your corporate website.” Today, all it takes is a few clicks to build a website. Currently, data science projects can feel a bit like a lock-in between data scientists, data engineers and domain expertsbut low-code / no-code technology holds the keys to a successful future for Enterprise AI.
6. Think and Act Responsibly
Before you adopt AI, you need to know what it is doing and how. You must ensure the software can communicate its decision-making process in a way that can be easily understood and scrutinised by business teams. Boards want this assurance and regulators will expect it. According to Girish,
“We need to collaborate more with the regulators. For example, if I start launching my edge AI models on the chainsaw, which is not only self-learning but also taking decisions on the control system itself, it can be dangerous and could start killing people. Cars have gotten better and better, yet the rules have gotten stricter and stricter. If we do not have those rules, it will be a wild west.”
For more information on the responsible and ethical use of AI, check out the ‘Future Says…Ethical AI’ episode with Aiko Yamashita, Senior Data Scientist Centre of Excellence at DNB Bank.
Conclusion
McKinsey estimates that AI will add $13 trillion to the global economy over the next decade, yet companies are still struggling to scale up their AI efforts. It is critical that digital transformation is viewed as a strategic imperative rather than just another technological trend. While your AI strategy will evolve, the focus should be on a culture of learning and continuous progress. It should be driven by your business objectives, not the other way around. These are not minor steps, but as AI continues to accelerate across industries, becoming an AI-fuelled organisation will be more than a strategy for success – it will be mission critical for survival. Remember to start small and scale fast. Pick leaders who can drive this enterprise-wide in a responsible manner. Choose partners who can grow with your ambitions.
Finally, if you want to see this interview in full, as well as all upcoming episodes in Altair’s ‘Future Says’ Series, please follow this link: altair.com/futuresays
About the author
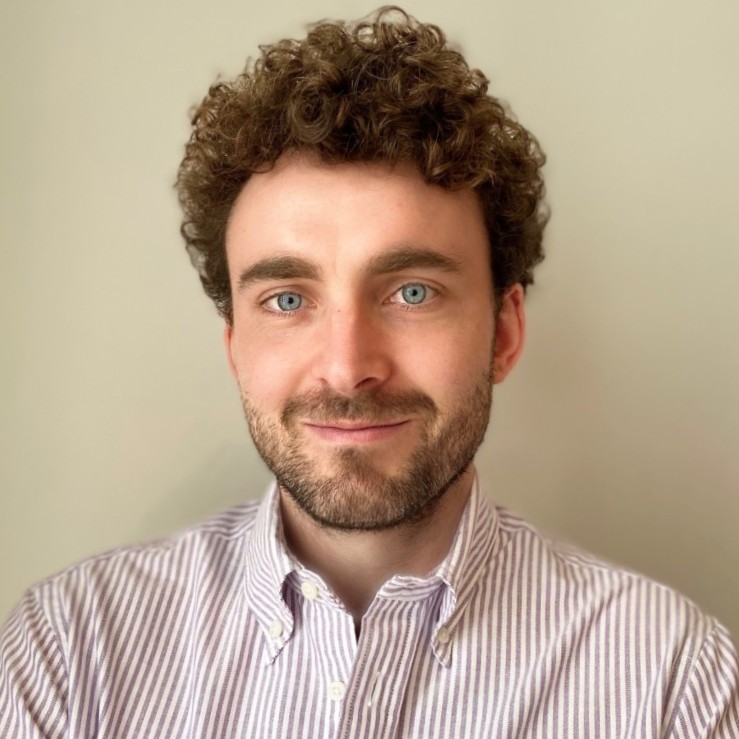
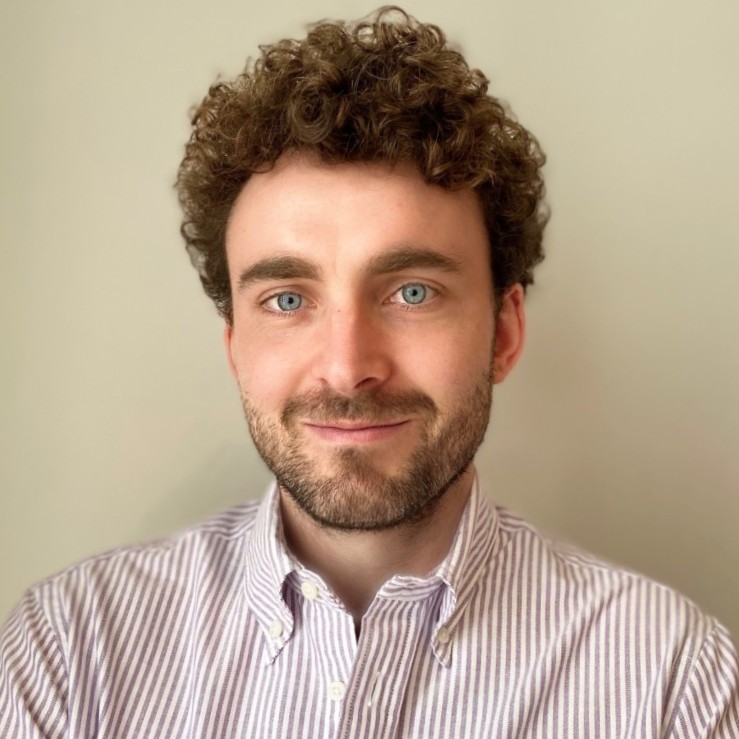
Sean Lang is the founder of Future Says – an interview series where he debates the pressing trends in Artificial Intelligence alongside some of Europe’s leading voices in the field. At Altair, Sean is helping educate colleagues and clients on the convergence between engineering and data science. From his background at both Altair and Kx Systems, Sean is passionate about championing data literacy and data democratisation throughout the enterprise. He believes in a future where everybody can consider themselves a data scientist.
Add comment