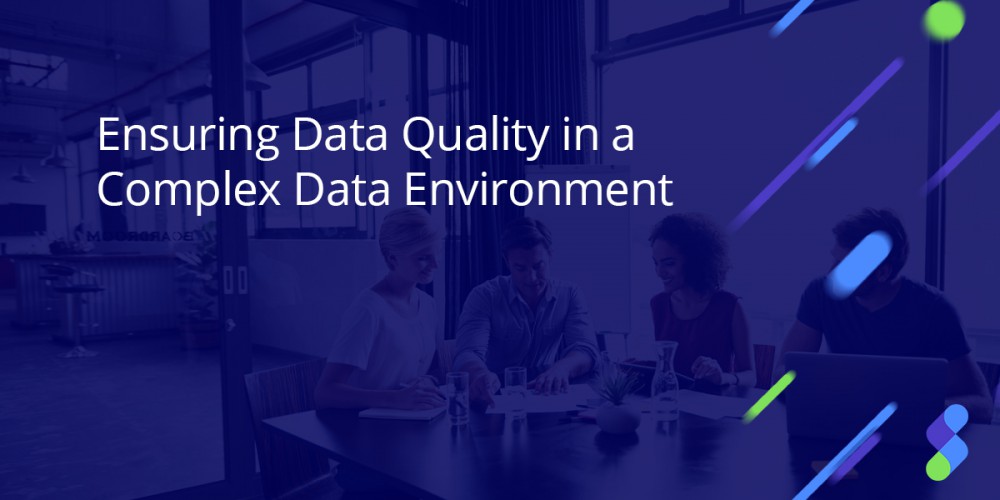
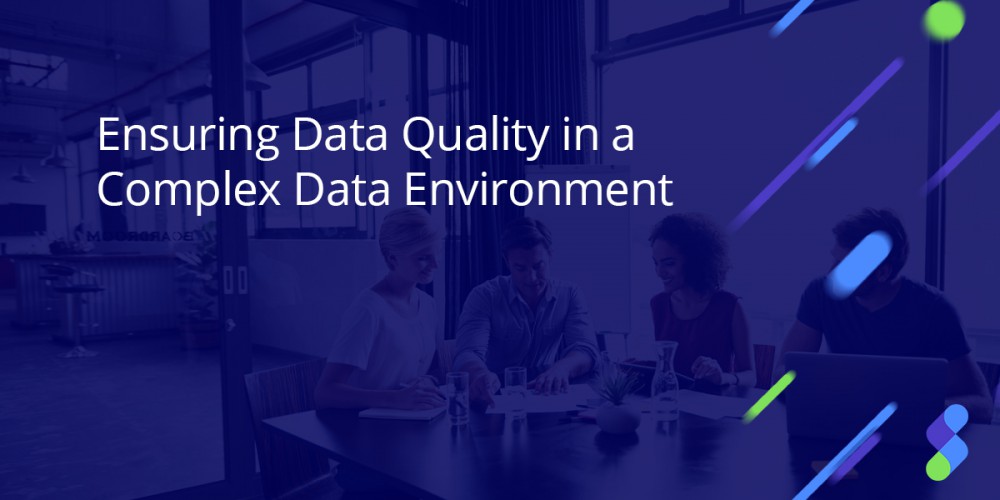
Data quality is considered as the highest commandment in data management. And it’s with a strong purpose. Only data of high quality is useful data, and to be quality it must be consistent and unambiguous. All data that is gathered, stored and consumed during business processes directly impacts the success of the business.
But as the amount of data and the number of data sources are exponentially growing, ensuring data quality across the entire enterprise is getting extremely complex. Companies are facing considerable challenges when it comes to maintaining a good quality of data. For this purpose, we invited Harald Smith, formerly Director of Product Marketing at Syncsort, to discuss the current state of data quality, common pitfalls, connecting with emerging tech and data governance and best practices that can help companies in their efforts to ensure quality data in their enterprise.
Hyperight: Let’s start by introducing yourself and telling us a bit about your background and Syncsort’s area of expertise.
Harald: I’m Harald Smith, Director of Product Marketing at Syncsort, where I’m responsible for strategy around Syncsort’s market-leading enterprise data quality and integration software solutions. Syncsort’s products provide a simple way for organisations to optimize, assure, integrate and advance data, with a particular emphasis on helping enterprises prepare data (including from legacy systems) for next-generation platforms and analytics.
My background includes experience in product management and solution architecture with a focus on accelerating customer value in the integration, management, and use of information. I also have extensive expertise in product and project management, data quality products and solutions, application development including Agile methodology and UX design, technical services, and business processes.
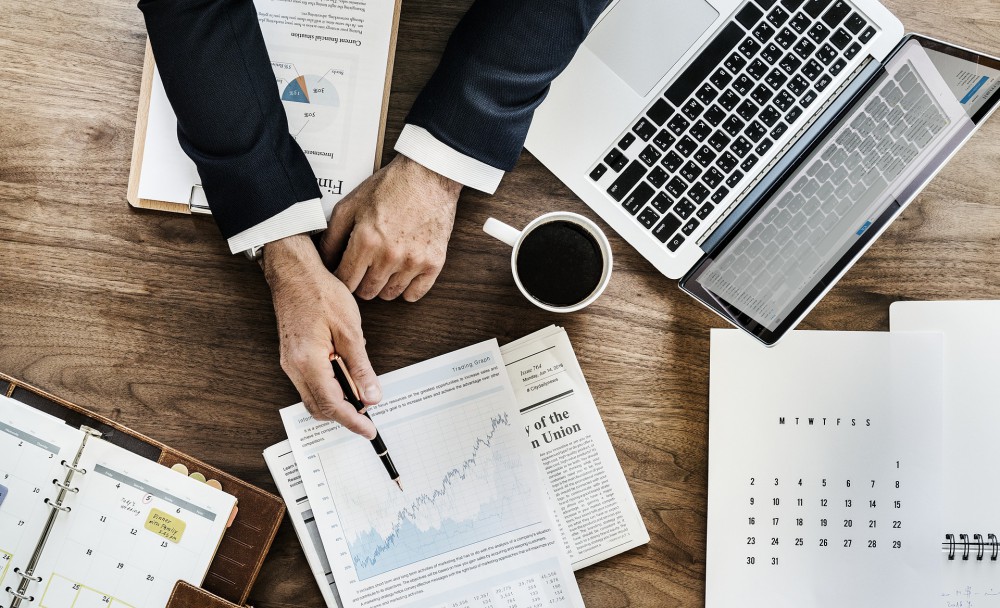
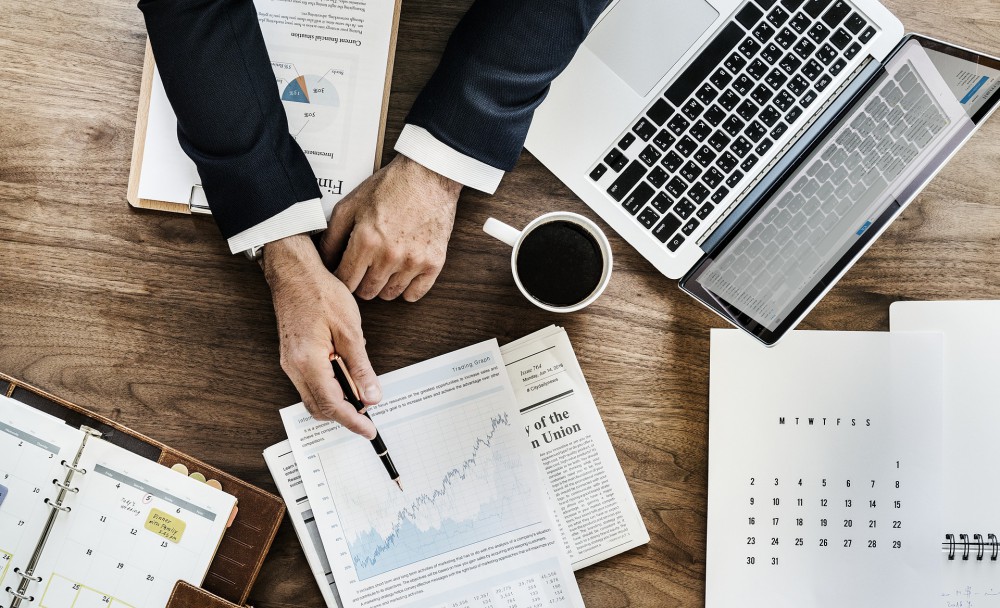
Hyperight: The amount of data that is generated and gathered in companies is increasing at an unprecedented pace. From your perspective, how much are companies successful in maintaining a high level of data quality in their enterprise and are they investing enough in it?
Harald: From my perspective, by and large, they are not. Collecting data from diverse and high volume sets of data means it becomes more difficult to ensure the data is high quality and also increases the difficulty in ensuring that the data is “fit for purpose”. As enterprises move towards real-world machine learning and artificial intelligence use cases, we’ll see more mature conversations about data quality happening as companies get serious about its impact.
Poor quality of data can cost a business an average of $15 million a year, according to Gartner, but the impact is becoming an even bigger risk as machine learning or artificial intelligence multiplies the impact exponentially. As we’re seeing increasing shifts to next-gen platforms like cloud, we’re also seeing more data quality issues emerge.
A new survey indicates nearly 80 percent of AI and machine learning projects have stalled due to issues with data quality and proper labelling of data attributes. This survey also noted two-thirds of respondents cited “bias or errors in the data” as a common issue and half reported, “data not in a usable form.” And then we fall into the classic “garbage in, garbage” out the reality of poor data quality, wherein moving the data to these new platforms (like blockchain and cloud) and replicating it further simply propagates the issues further along the data pipeline in more and more instances.
Hyperight: Let’s discuss data quality and emerging tech – what are the pitfalls? Are there any special considerations for data quality and emerging tech adoption?
Harald: Data quality has rarely been black or white – there’s always a contextual aspect to data. But for emerging technologies, there are new considerations that come into play. Current data quality solutions are being stretched as data has to be evaluated, integrated, de-duplicated, cleansed and matched across a variety of data sources in preparation for more advanced use cases like predicting customer behaviour, analysing risk or detecting fraud which requires analysing huge volumes. At the same time, issues such as bias and provenance are emerging that make it harder to discern whether certain data sources are even usable or valuable.
Emerging use cases such as machine learning or blockchain have new or different requirements and needs. Consider how simple, obvious correlations existent in data might become the “insight” that machine learning finds. Or how the immutability of blockchain may propagate a data quality issue that would otherwise be updated and resolved.
To solve the challenge, companies must make an ongoing commitment, which begins with creating an overarching strategy that puts data governance and data quality best practices front and centre, including the compliance requirements and the different data quality measures needed for different use cases, purposes and outcomes, before developing projects. Also, consider the ethical and corporate implications of these business initiatives. What happens if the AI and machine learning models receive biased, dirty, or inaccurate data? Biased or polluted outcomes could be lethal for an enterprise’s brand reputation, revenue growth or compliance requirements.
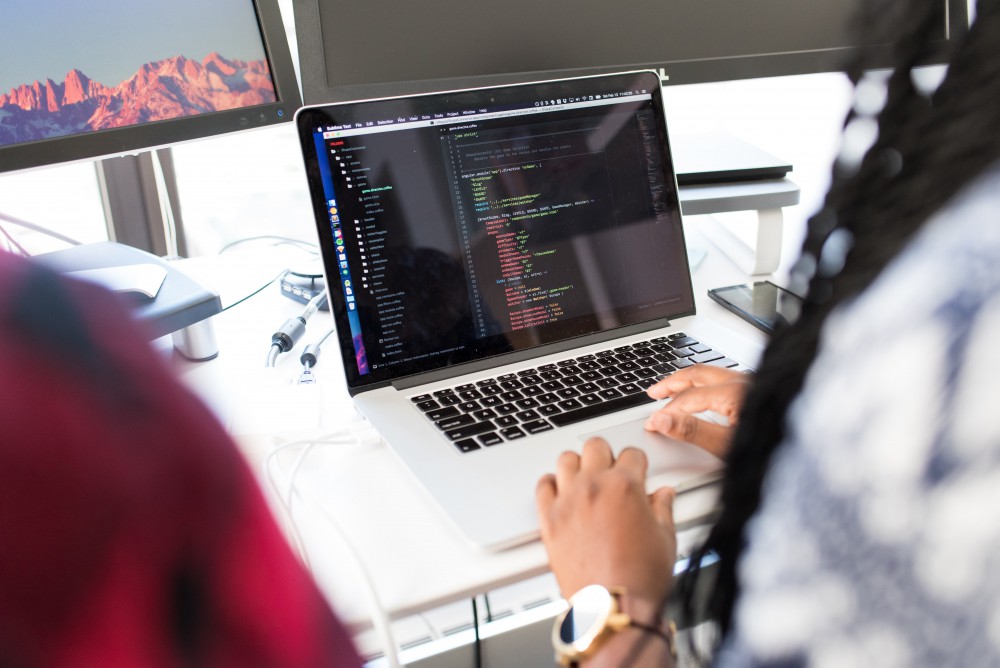
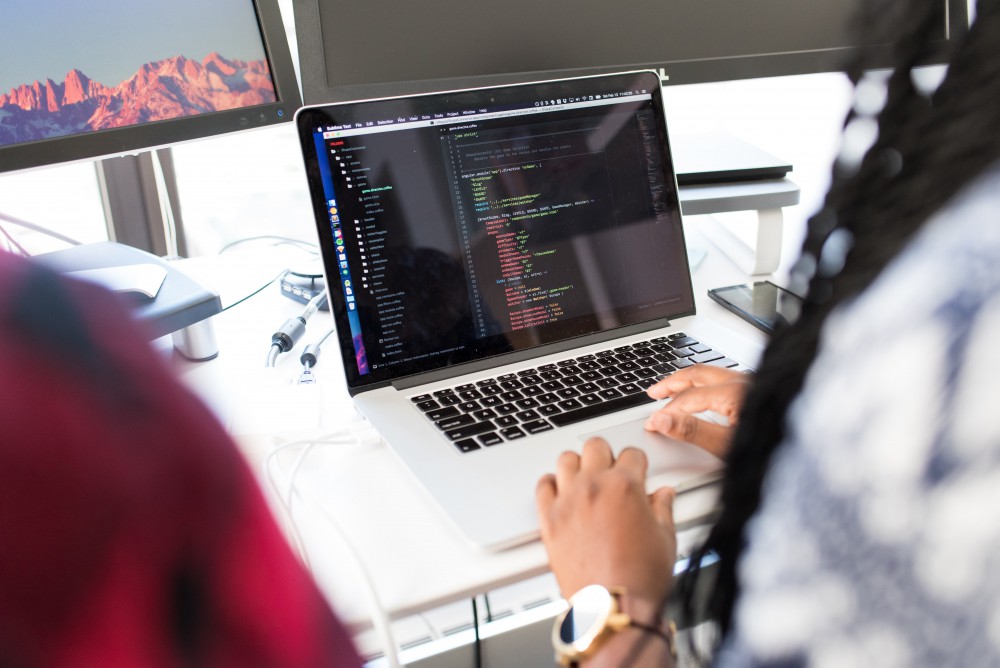
Hyperight: How can ML tools help manage Data Quality?
Harald: Machine learning has an important role to play in data quality, particularly to address the limitations of manual review in an effective period of time. Given the high volumes and ever-increasing variety of data all enterprises are dealing with now, humans simply do not have the time to assess all data and monitor it continuously for variation. We might well notice when a sensor fails or a data quality metric fails to meet a threshold and an alert is triggered. But what about when false data is injected into a sensor, or unexpected duplicates are passed through? A computer program can scan vast volumes of data in mere minutes or hours and evaluate it according to your needs, whether finding missing, mismatched or duplicative entries or uncovering new and often unexpected correlations.
However, you can only take advantage of these tools when you understand the business problems and processes, as well as the data requirements and data available to help address those problems – not to mention put in place the people who understand the data and its context, and the processes to help these people govern, validate, vet, and leverage that data.
Hyperight: What are some best practices for achieving data quality?
Harald: First and foremost, you need to think continuously about the business problems you are trying to solve. This is a central, operating best practice that helps make the organisation data literate and ensures everyone has a clear understanding of how they need to approach the analysis and use of data to meet enterprise needs.
Using the right tools is a crucial part, but equally important is human understanding of the data so that crucial judgement can be applied. Knowing what data exists isn’t enough; context around how it’s used becomes key, because a set of data that works just fine for one function does not necessarily mean it can be used for a different one. Particularly in cases where data is in specific silos within an organisation (or outside it if bringing in other third-party data), you need enough knowledgeable people about how to use and take advantage of the tools at hand to investigate and analyse data, often in ways that may not be immediately obvious. This depends on establishing and valuing a data-literate culture including people, process, and tools. And these three elements do not exist in isolation.
To ensure people become data-literate, they must be included in processes and communications that help them become informed and help them share what they know and have learned. Building out a “library” of knowledge about data, processes and approaches for using the tools at hand for data analysis is central to developing a data-literate culture.
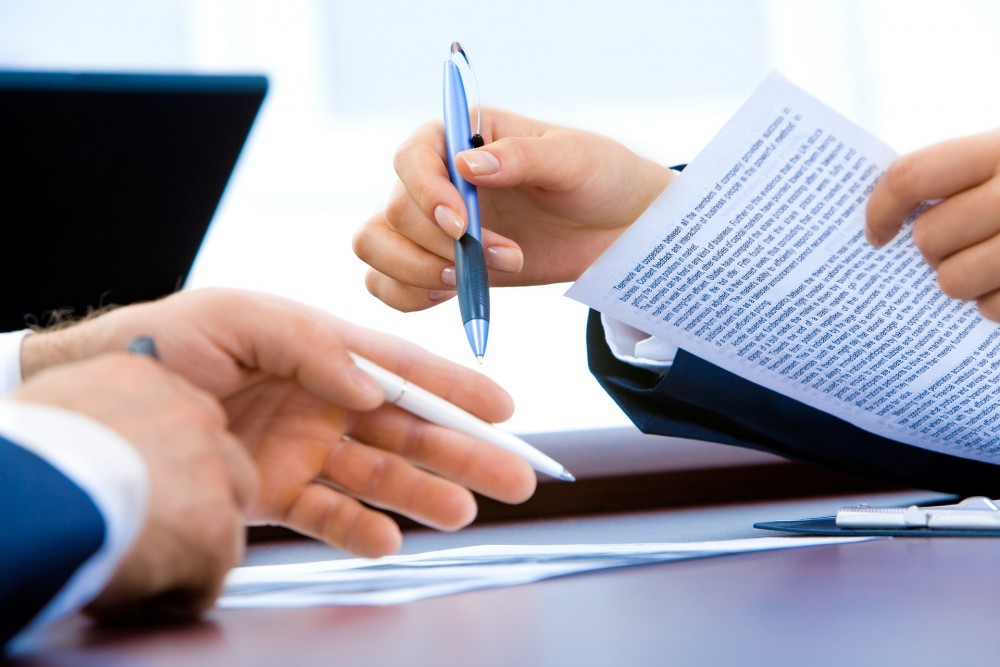
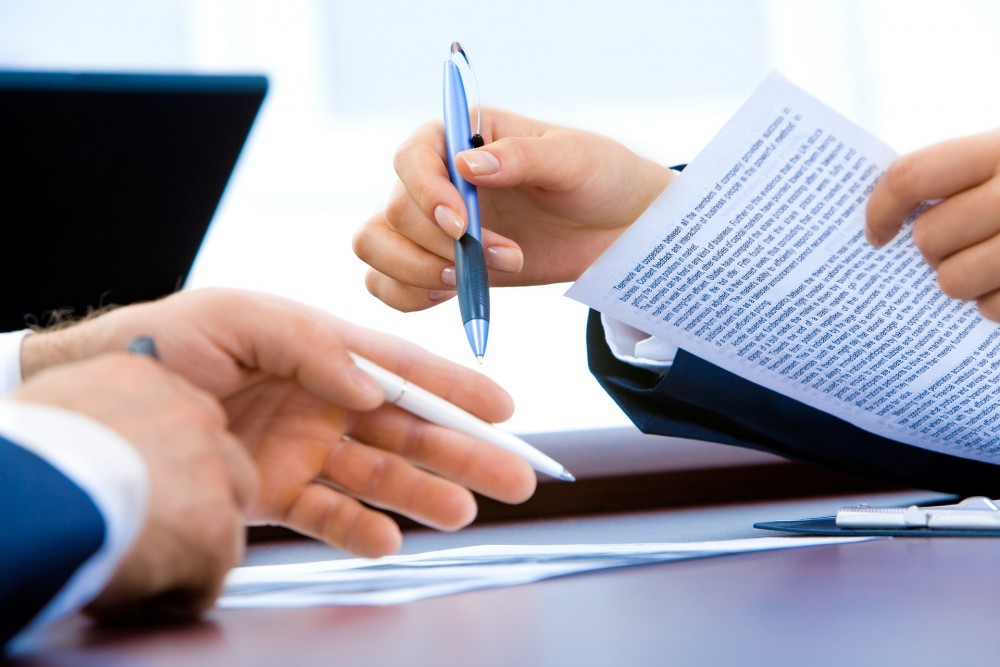
Hyperight: What’s the relationship between data governance and data quality?
Harald: There’s a symbiotic relationship between data governance and data quality. Many people think of data governance in terms of how data should be archived, ensuring its security and that it was compliant with any laws or regulations. Of course, these are important components of a data governance strategy and process, but there’s been a shift in data governance thinking to fully embrace it as the overarching aspect of a data strategy, where it incorporates how data is used to facilitate accessibility, drive revenue and even monetise data.
That strategy will only be successful with strong data quality measures in place. Where data governance defines the framework for what data is relevant and pertinent to the business needs, and why; data quality provides the practices that implement the rules of that framework and measures the compliance of data against that in order to help compile the best standards and monitor the quality of the data over time. That continuous iterative cycle is what helps generate trust in data – trust that it can be leveraged effectively for the initiatives the organisation has in mind to increase revenue, reduce risk, meet compliance, and reduce costs.
Find out what’s the state of enterprise data quality today in Syncsort’s extensive survey that covers challenges and opportunities for companies that are trying to bring data quality across the entire enterprise.
Add comment