Machine learning is transforming every aspect of advertising. In the era where social media advertising is the primary advertising for brands, the tech giants like Twitter are harnessing ML to help brands in every step of advertising, from ad creation, testing creative variations, improving performance, to audience targeting to ad buying and optimising spend. To learn first hand how to utilise ML in ads, we talked to Nastaran Ghadar, Engineering Manager at Twitter, which is also her session topic at the upcoming NDSML Summit 2021. Nastaran also shared her experience with working at Twitter, provided a sneak peek into what a machine learning model factory is (which you’ll be able to hear more about during the panel Nastaran will be leading at the NDSML Summit), and machine learning trends we’ll see in 2021 and beyond.
Learn more about the NDSML Summit
Nastaran will talk more depth on this topic in her NDSML Summit session on general techniques around how you can use ML to create better predictions in ads impressions and also how data can be applied in targeting different user demographics and what are some of the technologies commonly used in this area.
Hyperight: Hi Nastaran, welcome to the NDSML Summit 2021. We are super excited to have you! To start off, please share with us a little bit more about yourself and your background.
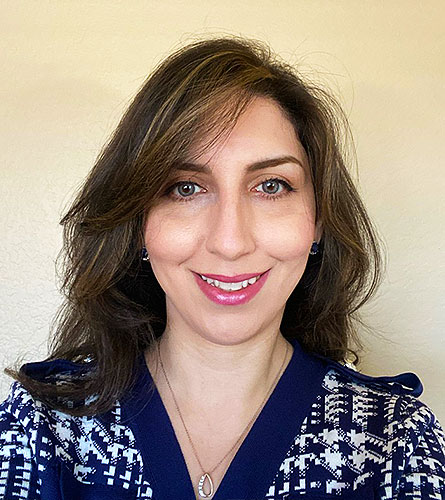
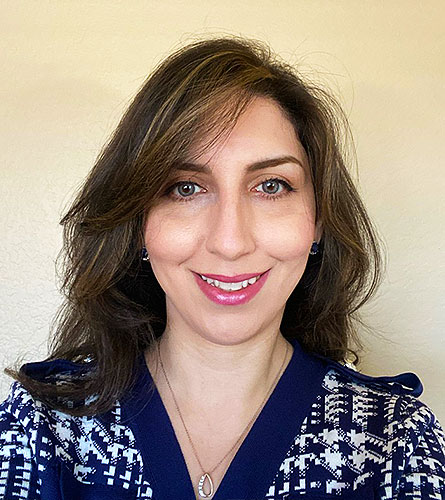
Nastaran Ghadar: Hello, thank you for having me! Happy to be here with you! A little bit about me; I have a Ph.D. in computer engineering from Northeastern University with a focus on Machine Learning and Computer vision. I have a passion for building products that change the way we live, by directing an end to end designs from concept to market.
I have been in the field of AI and ML for more than 15 years now and am still fascinated by how much it has shaped our modern technology in every aspect. During this time, I have led and worked on several projects in a wide range of technology applications such as biomedical engineering, animal behaviour analysis, drones, industrial robotics, self-driving cars, ads, and social media. Currently, I am an engineering manager at Twitter leading a group of very talented ML engineers on core features and foundational modelling challenges in the ads domain. Prior to joining Twitter, I have worked at other tech companies such as Lyft, ABB, and Draper.
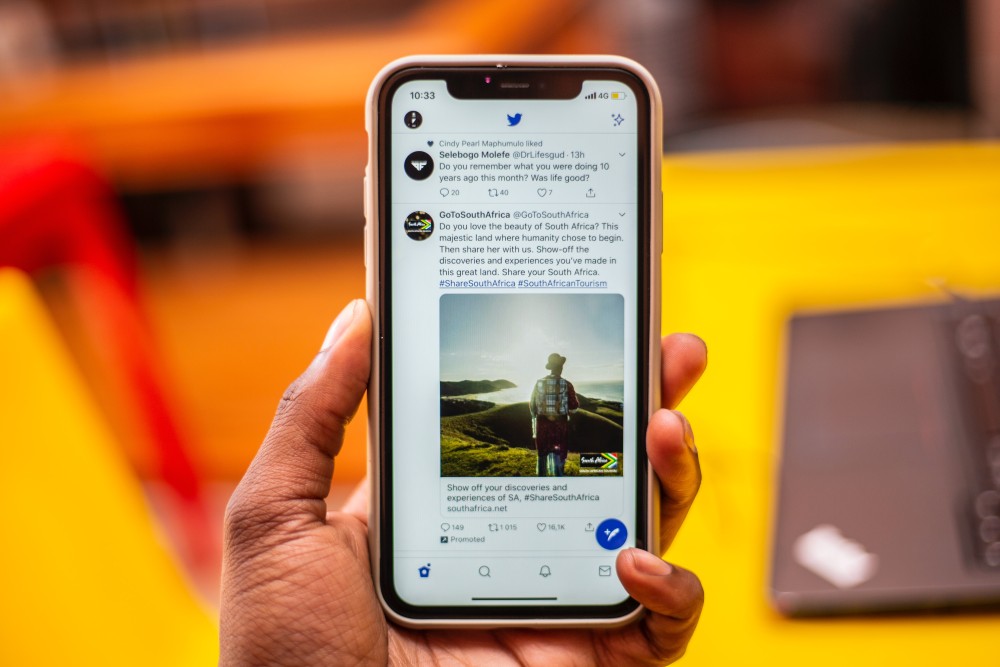
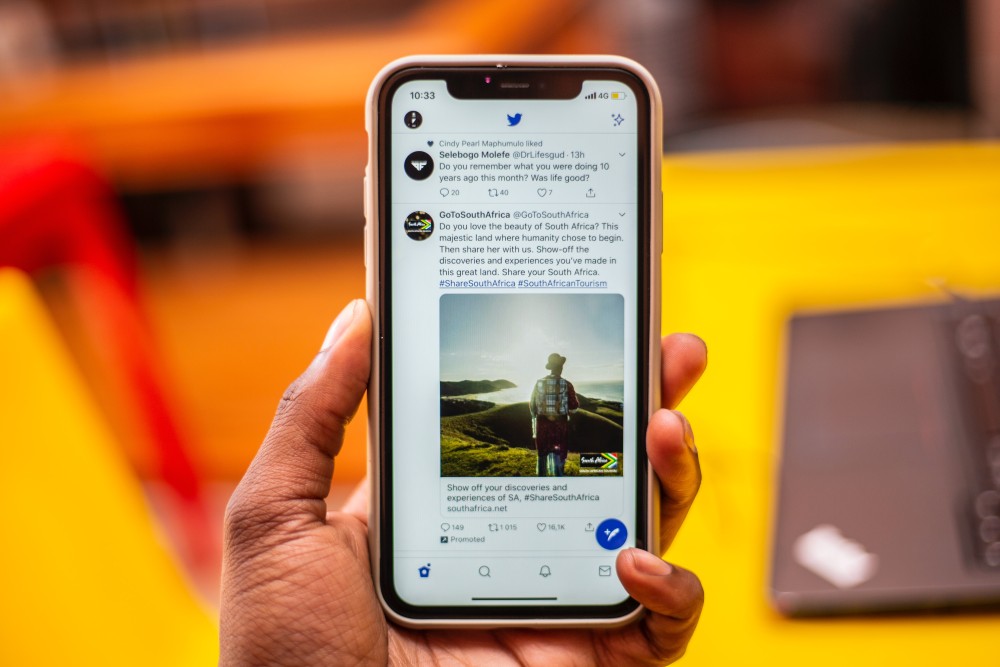
Hyperight: Your NDSML Summit 2021 session will focus on How to utilize ML in Ads. This is a particularly appealing topic for advertisers and marketers looking to create super-targeted campaigns. What are the opportunities of utilising ML in advertising and marketing?
Nastaran Ghadar: There are several stages in the advertising and marketing funnel where we can use AI and Machine Learning such as better targeting of users and customers for specific campaigns and ads, or curating better and more relevant content for different audiences. The logic is simple, basically, find users’ interests by understanding some basic features such as age demographic, and location, then feed this information to an ML model to predict whether a certain type of user would be interested in the Ad. Or another approach is to find users with similar taste and assume that often they enjoy similar content or ads. So if user A is interested in a certain Ad, and user B has similar features compared to user A, most likely user B is a good audience for that Ad as well. User features such as age, gender, location, etc., are great indications of what they would be interested in or how to group users together to better understand what they are looking for.
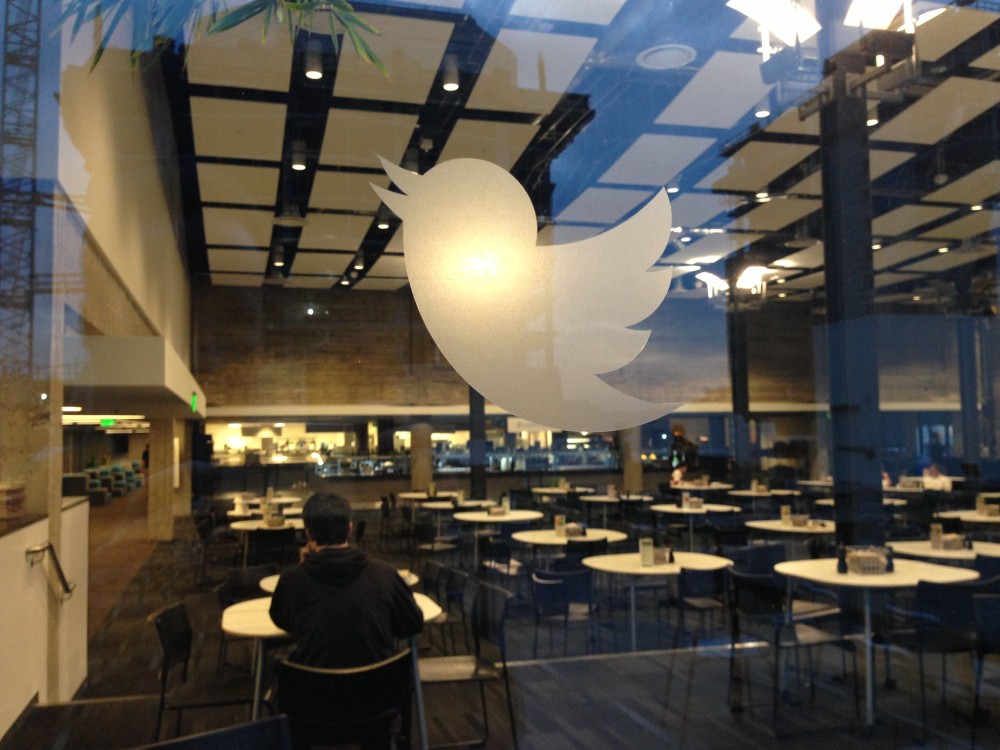
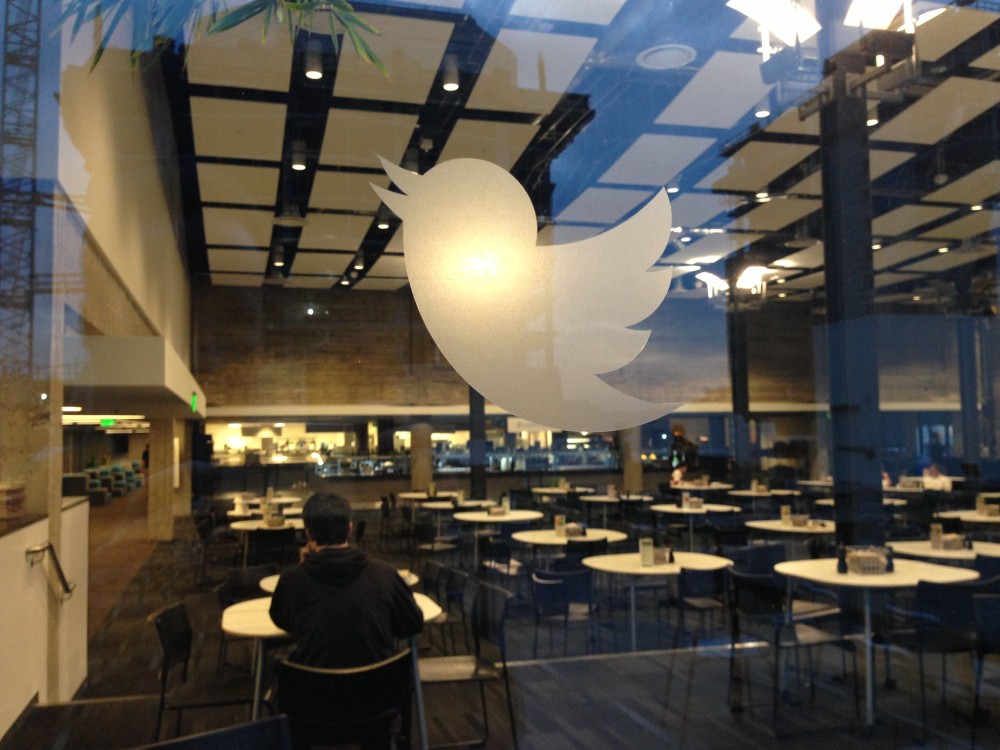
Hyperight: What have been your most significant learnings as an Engineering Manager at Twitter?
Nastaran Ghadar: Twitter is a great place to be at and leading an engineering team at Twitter has taught me a lot. I have learnt that driving a successful campaign comes from a variety of reasons, such as excellence in engineering work, great tech and analytics tools, but most important of all is to put yourself in the shoes of both advertisers and users to understand what makes sense to them and find those specs where it can be unique for their mutual benefit. Once you have a clear picture, you can work with engineering tools to create models and generate features and metrics that best suit them and run a successful business with having both the client and users in mind.
Hyperight: One of the biggest struggles in the AI field is the AI talent shortage. How can this challenge be solved or mitigated?
Nastaran Ghadar: I believe that we should start exposing the new generation from early stages in their education to AI and ML. The world is changing every day and to keep up with it, we need to constantly teach ourselves about the new technologies and findings in different areas. In the meantime, since AI is literally everywhere, reading short articles or taking online courses to understand the general concepts and new findings can be a great step towards closing the gap between demand and supply.
I have seen folks that graduated several years ago from college with no AI background, self-learning different applications in AI depending on their interests and being able to apply them to their current roles. If you are interested in working on AI, and have no prior experience, you shouldn’t be afraid to start learning and using it. Just start simple, pick a side project, use many of the available online resources and build an end to end pipeline. After finishing a few examples, you can also use them in your daily job.
Hyperight: You will also be leading a panel on the topic of Trends and hypes in Machine Learning Model Factories & Management. Could you please share what a Machine Learning Model Factory means and what the benefits are for ML deployment?
Nastaran Ghadar: Basically a machine learning model factory is a system that can create prediction models automatically with minimal manual work. There might be several modules in an ML factory, such as a training module, learning module, or deploying module. Depending on the application of each module it might be quite complex with several layers or simple. Imagine you have several hundred consumers with unique needs and requirements, now imagine if you were to manually train a model for each consumer separately. At some point, scaling becomes impossible and the only way we can actually deploy thousands of models at once is to have decent automation in our processes just like every literal factory.
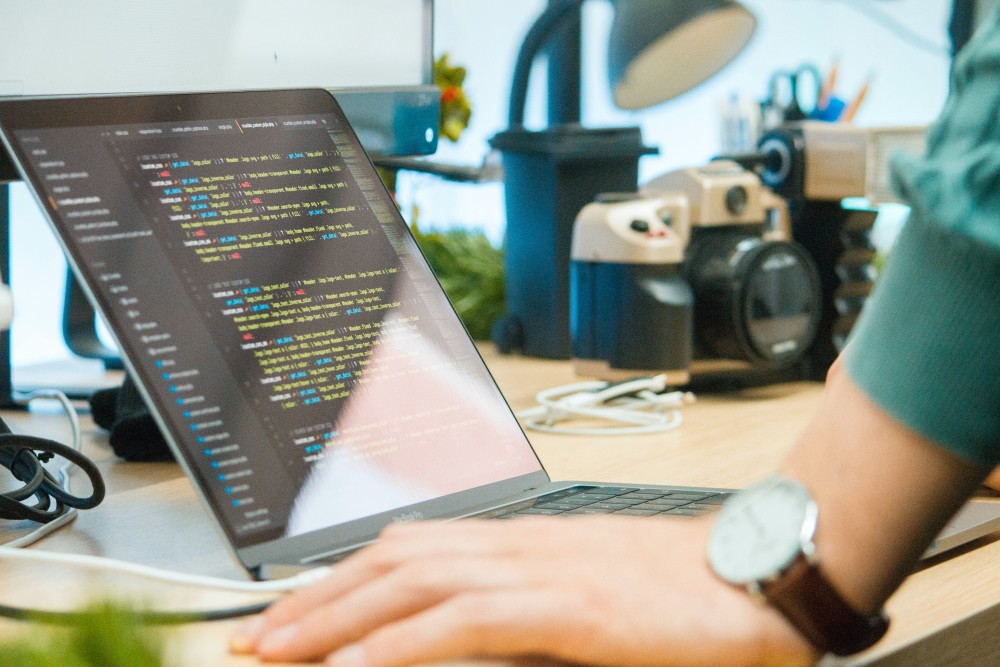
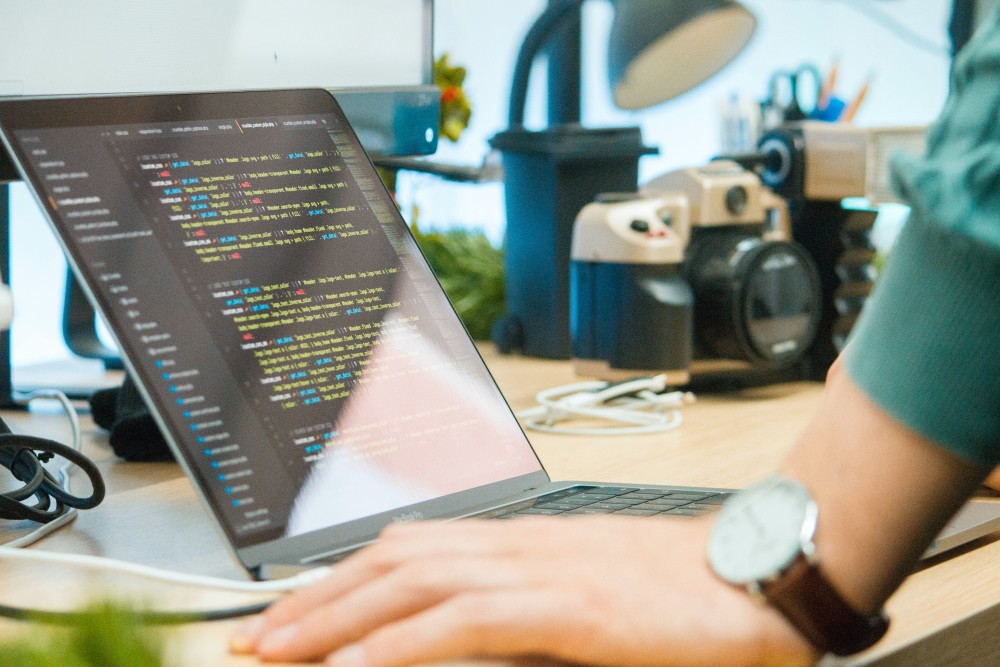
Hyperight: And finally, what other trends in machine learning do you see taking shape in 2021 and beyond?
Nastaran Ghadar: I think this year due to COVID, there are some trends that a lot of folks are investing in. Many industries and factories took a hard hit and it became a necessity to have all the way smart systems where not only you can build smarter but also you can make intelligent decisions such as predicting when supply chains may get disrupted or when downtime may happen. Another strong trend is how to use AI to ease and improve work from home experience and one hot trend is around augmented reality and improvements around video/audio/text understanding (such as utilising computer vision, natural language processing). Robotics and self-driving are still going strong (such as the use of reinforcement learning and smart simulations) and the need is even more during this pandemic. E-commerce and Social media applications also are going very strong as the number of users is growing rapidly and having a better experience is key.
Add comment