Data-driven thinking is important for every organization. However, the decision-making process can be difficult for growth companies that lack data. Managers see this as an obstacle when it comes to predicting the company’s future revenue and pushing the business to the next level. At the same time, investors also have a challenging time when making thoughtful and informed decisions for investing due to the lack of data and a prediction model of the revenue of the private companies in a high-growth stage.
The experts from EQT Motherbrain found a solution on how to use data points from other companies to develop a method that can be helpful for growth companies to address the issues with limited datasets when predicting revenue.
This method is called SiRE (Simulation-Informed Revenue Extrapolation). It not only replaces the traditional way of revenue modelling, but also it is a tool to build trust around forecasts. Let’s delve deeper into the benefits SiRE offers to private growth companies, as we hear from two experts: Lele Cao, Staff Data Scientist and Motherbrain DS/ML Lead at EQT Partners, and Vilhelm von Ehrenheim, Principal Engineer and Motherbrain Lead at EQT Partners.
If the insights they will uncover in this interview are interesting for you, then you must follow their presentation at this edition of the NDSML Summit, or sign up for Hyperight Premium to access their talk after the event.
Hyperight: Can you tell us more about you? What are your professional background and current working focus?
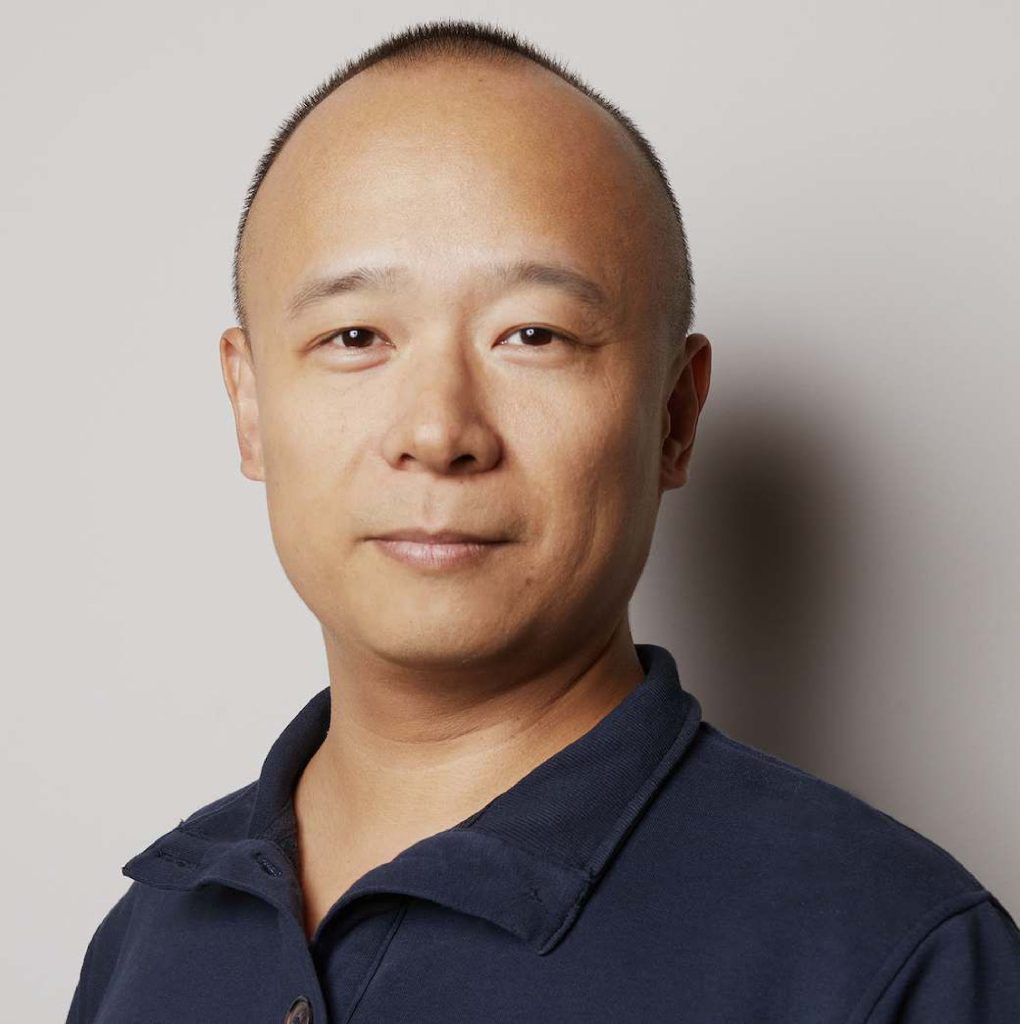
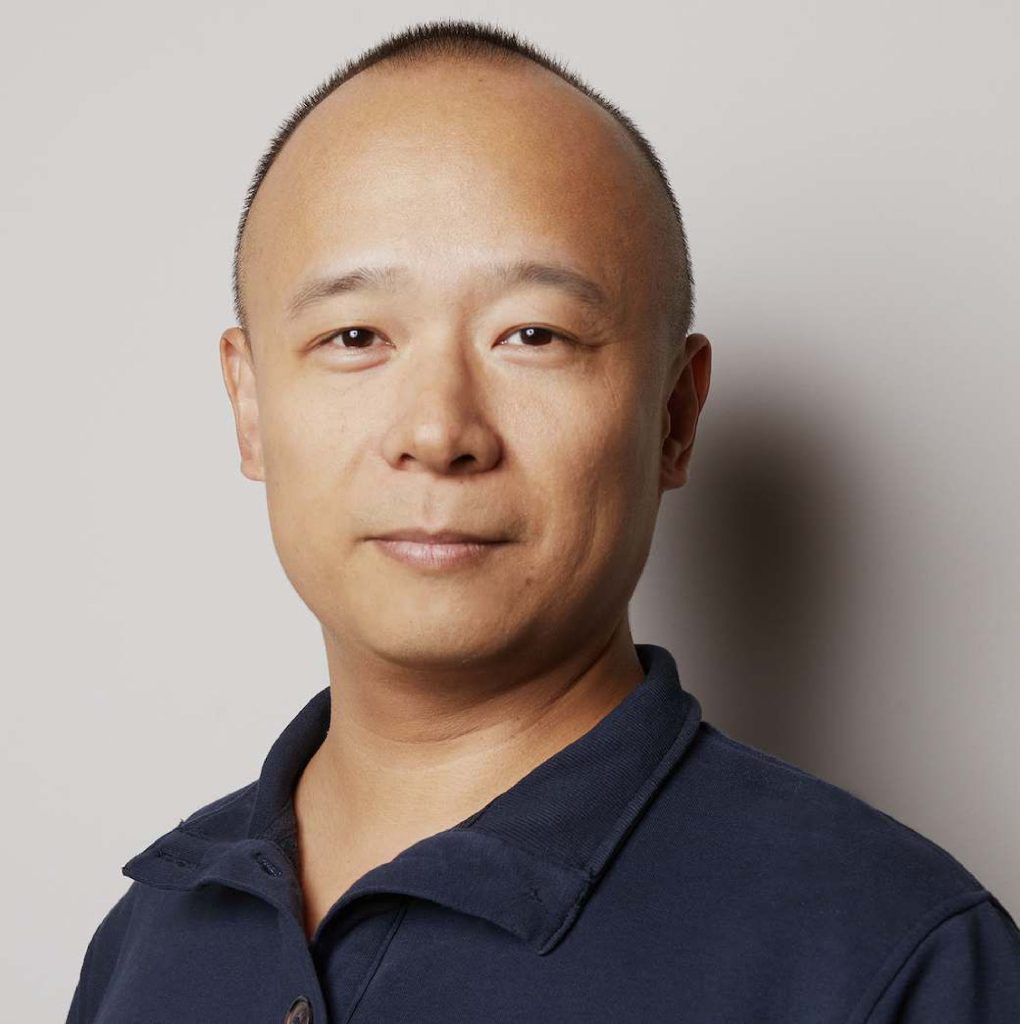
Lele Cao: I’m currently a staff research scientist in EQT Motherbrain. I mainly lead the research effort around solving the key and common problems faced by our investment professionals, using Machine Learning methodologies. Prior to EQT, I had been working on many different Machine Learning projects in King and Alibaba. My Ph.D. research started in 2012 and was about robotics and information fusion. Before 2012, I had more than 7-year software engineering experience in the telecom sector.
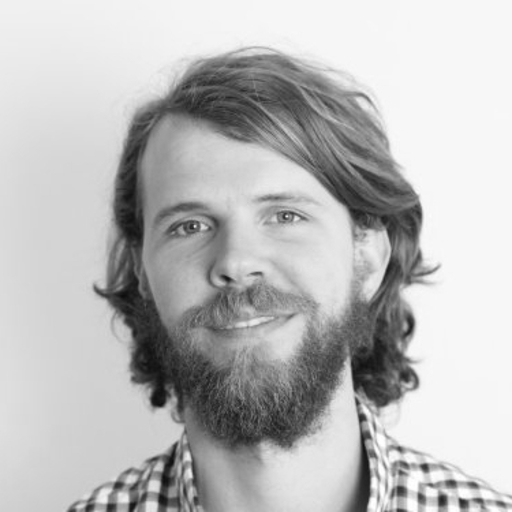
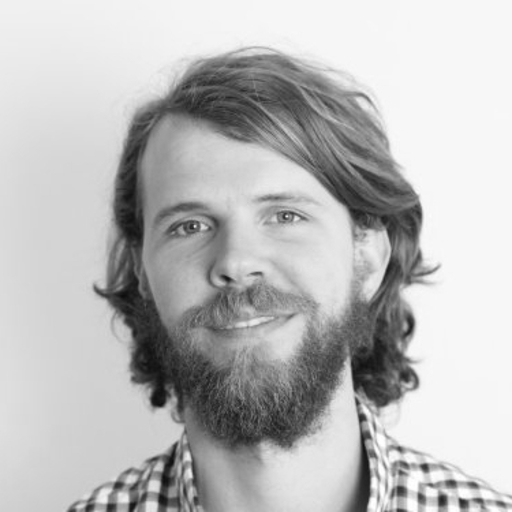
Vilhelm von Ehrenheim: I have spent the last 5 years building out the Motherbrain platform at EQT, leveraging Machine Learning and data to make EQT an ever smarter investor. During this time the team has grown from 3 to 30 persons, and Motherbrain has become the central platform for tracking deal flow across multiple EQT business lines. Before EQT, I was building production Machine Learning systems at Klarna enabling real time decisions for credit and fraud risk.
Hyperight: During this year’s NDSML Summit, you will share more on “Revenue Forecast for Growth Companies Using Scarce Time-Series Data”. What can the delegates at the event expect from your presentation?
Lele & Vilhelm: Our presentation is going to demonstrate how one can predict a company’s future revenue using a very limited amount of revenue data points from other companies. The method is abbreviated SiRE – Simulation-Informed Revenue Extrapolation. This is one of the many scenarios where deep learning is not easily applicable due to factors such as lack-of-data and explainability demand.
More generally, this approach might be suited to perform time-series extrapolation on a small dataset. We publish a paper and the source code so that practitioners can quickly reproduce the results and try SiRE on their own use cases.
Hyperight: If we understood correctly, the presentation is based on a research paper that you and your colleagues recently published, and that will be presented at the 31st ACM International Conference on Information and Knowledge Management (CIKM). Congratulations! Can you tell us a bit about the paper? What was the main hypothesis, how did you come up with the topic, and who else was involved?
Lele & Vilhelm: Thank you. We are happy that our work is peer reviewed and recognized by the research community. CIKM is a top tier academic conference with a focus on Data Mining, Information Systems and Databases.
Accurately forecasting companies’ future revenues will provide valuable insights to management teams and owners. Investment professionals, including the ones from EQT, often rely on such revenue forecasts to approximate the valuation of private companies in a high-growth stage, and inform their investment decisions.
However, this task is usually manual and empirical, leaving the forecast quality heavily dependent on the investment professionals’ experiences and insights. Furthermore, financial data on scaleups is typically proprietary, costly and scarce, ruling out the wide adoption of deep learning approaches.
Our approach, SiRE, can work on small datasets based on the main assumption that revenue development likely repeats historical patterns for similar companies at similar stages.
The ideation, exploration, experimentation and implementation is carried out by EQT Motherbrain in conjunction with EQT Growth and EQT Ventures.
Hyperight: What are the current ways of performing revenue predictions when approximate the valuation of a company in a high-growth stage, and how does the proposed model help solve any challenges when doing these predictions?
Lele & Vilhelm: As we mentioned previously, the current way is mostly manual and heavily rely on individuals’ experiences and insights due to lack of data and requirements of simplicity and explainability.
Concretely, one can choose to either trust the management case or work on their own model. The management case is merely the forecast of the company’s management team. When it comes to creating one’s own forecasting model, there is tremendous space for customization.
In general, practitioners aim to create different cases (e.g. base, high and low cases) by varying a limited number of factors such as market structures, competitive situations and benchmarks. Most of the time, strong assumptions are made about the operating model, based on which the revenue data points are recursively calculated into the future. In rare cases, a more complex model like ARIMA is adopted.
You might have already realized that the level of “correctness” is critical, yet hard to prove. Our method allows “hypothesis mining” from scarce data, leading to data-driven predictions that capture repeated patterns better.
Hyperight: One of the main challenges in setting such revenue predictions is data scarcity. But your model seems to be based upon that. Can you tell us more about the model, how it is built, and how confident one can be in its accuracy?
Lele & Vilhelm: Imagine how many reference companies a human will use to predict the next revenue data point? Probably not more than five, empirically. As a result, we try to replicate that process by asking the algorithm to look at data points from similar companies at a similar development stage.
Human brain does not have a rigid and structured way of distilling prediction from past observations, right? Why should the algorithm? Therefore, we randomly sample from similar data points and fit a prior distribution to the sampled points, naturally resulting in a confidence estimate from the fitted distribution. Confidence estimation provides investment professionals with guidance on the certainty of the outcome.
Of course, noises arise from data and sampling, we apply some denoising and smoothing techniques to obtain more robust predictions. Of course, this is a very high-level description of SiRE, please watch our presentation at NDSML for more explanations.
Hyperight: One of the benefits for organisations with the proposed solution and other data-driven forecasting techniques is making informed investment decisions. What else can they gain by unlocking possibilities of automating long-term generated revenue extrapolation using scarce data?
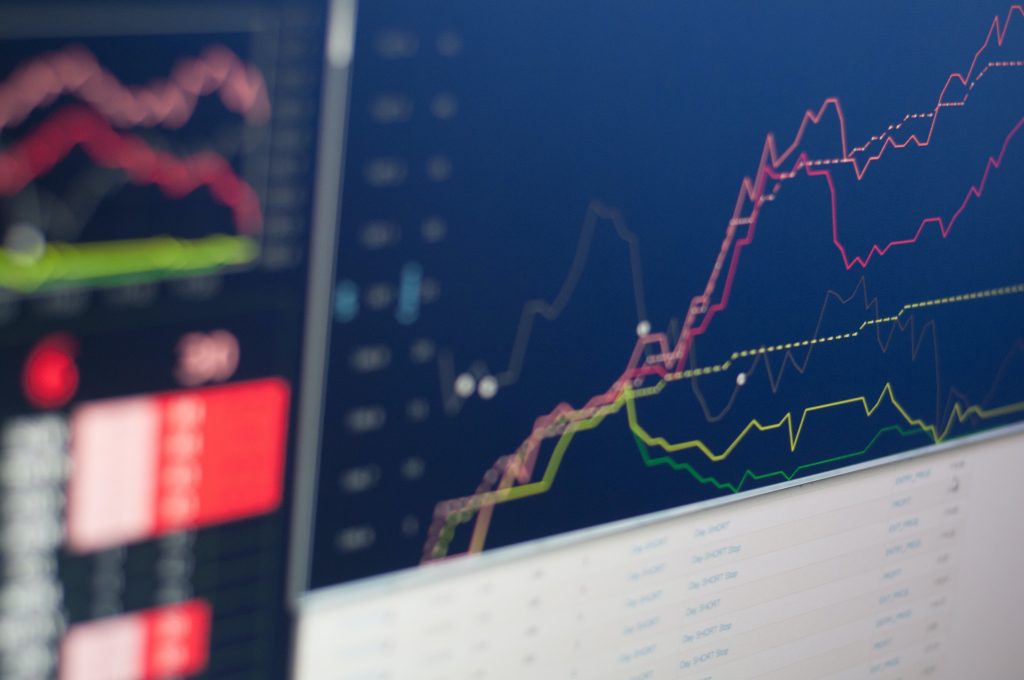
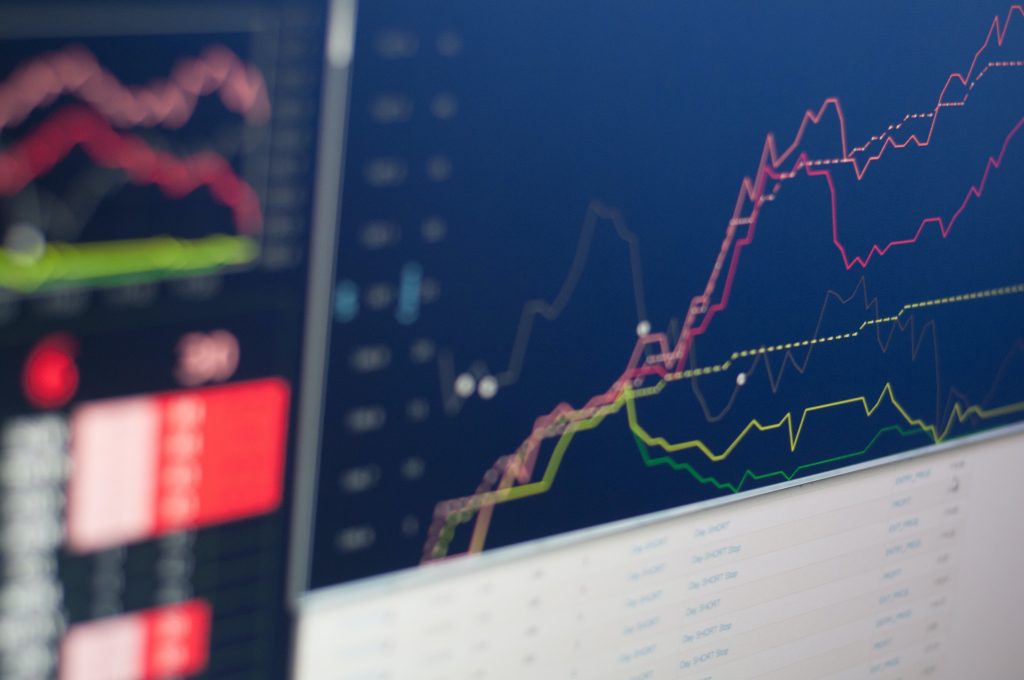
Lele & Vilhelm: Three years is a typical investment period in private markets. By providing such long-term and fine-grained forecasts, investors and managers are equipped with a power tool to gain a much more objective view of revenue trajectories.
SiRE, by no means, will replace the traditional way of revenue modeling: rather a tool to cross validate the results, and build trust around the forecasts.
Even better, EQT professionals will automatically get updated predictions whenever the benchmark data is changed, even just a tiny little bit.
Hyperight: In your presentation, you will also talk about how you productionize the algorithm on your investment platform. Can we know more about this step in the process?
Lele & Vilhelm: EQT’s investment platform is called Motherbrain, which is built by a group of talented designers, engineers and scientists. Motherbrain is created to transform the digital way of thinking within the private capital industry, by leveraging Big Data and Machine Learning to give EQT a unique edge.
Motherbrain supports the tracking of company life-cycles, during which revenue tracking is certainly an important part. Motherbrain allows users to input many metrics such as revenue. Any change, such as adding/removing/correcting revenue data points, might change the forecasts for many companies.
As a result, this algorithm is deployed in a way that it automatically incorporates those changes and updates the prediction accordingly so that the deal professionals can be informed in a timely manner. The algorithm is a pretty simple one hence super easy to deploy and schedule, as long as it has access to Bigquery and BigTable.
Hyperight: Do you have any final advice and recommendations for organisations interested in utilising the solution you offered?
Lele & Vilhelm: As of technology and research, EQT Motherbrain believes in sharing and open sourcing, so feel free to read our paper and run our source code. We can be contacted for any problem/question you might encounter.
The most challenging part leading to a successful application of SiRE is to have a reasonable benchmarking dataset containing revenue data of companies. But the minimum requirement of that dataset is relatively easy to reach. Which we provide more details in the paper.
Hyperight: From your perspective, how do you see the future of agile algorithms and ML systems? Any trends you see more of in the upcoming 1 – 2 years?
Lele & Vilhelm: The status-quo of Machine Learning Engineering is somewhat comparable to the early days of Software Engineering. Currently, ML engineering needs a unique combination of skills to master well, yet this is, and will continue to be improved so that the entry bar of ML engineering will be lowered significantly. The popularity of MLOps is one of the many examples that will lead us there.
As a subcategory of Machine Learning, Deep Learning is undergoing an increasing adoption in real-world applications. The overall AI research community will continue to encourage sharing and open sourcing, therefore the algorithm itself will not be regarded as most companies. Proprietary and private small datasets can be valuable assets to many businesses; and using Machine Learning methods to generate insights will be a common demand.
As a result, we mainly see four trends. Firstly, easy-to-use tools and frameworks to enable more engineers leverage the power of AI. Secondly, more methods will be invented to lower the requirement dataset size. Thirdly, the absolute majority of data is unlabeled, hence calling for label efficient methods. Lastly, data privacy and model security will gain more emphasis in the coming years.
Add comment