In an interview, we sat down with Rajiv Shah, a seasoned Machine Learning Engineer with ten years of experience as a data scientist. Rajiv provides a glimpse into Hugging Face’s role in revolutionizing the open-source AI community. As a former key figure at Hugging Face, Rajiv focused on addressing complex challenges using open-source AI.
Rajiv will also present on “Evaluation Techniques for Large Language Models” at the upcoming Data Innovation Summit 2024. He aims to equip delegates with actionable ideas for building robust models. Moreover, Rajiv sheds light on Hugging Face’s journey, its expansion beyond natural language processing (NLP), and its vibrant open-source community. He also touches upon challenges in evaluating large language models (LLMs). Additionally, he discusses practical applications of AI in enterprise teams and emphasizes the crucial role of interpretability. The interview concludes with his insights into upcoming AI trends, providing a concise yet comprehensive exploration of Hugging Face’s impact.
Hyperight: Can you tell us more about yourself and your organization? What is your professional background and current working focus?
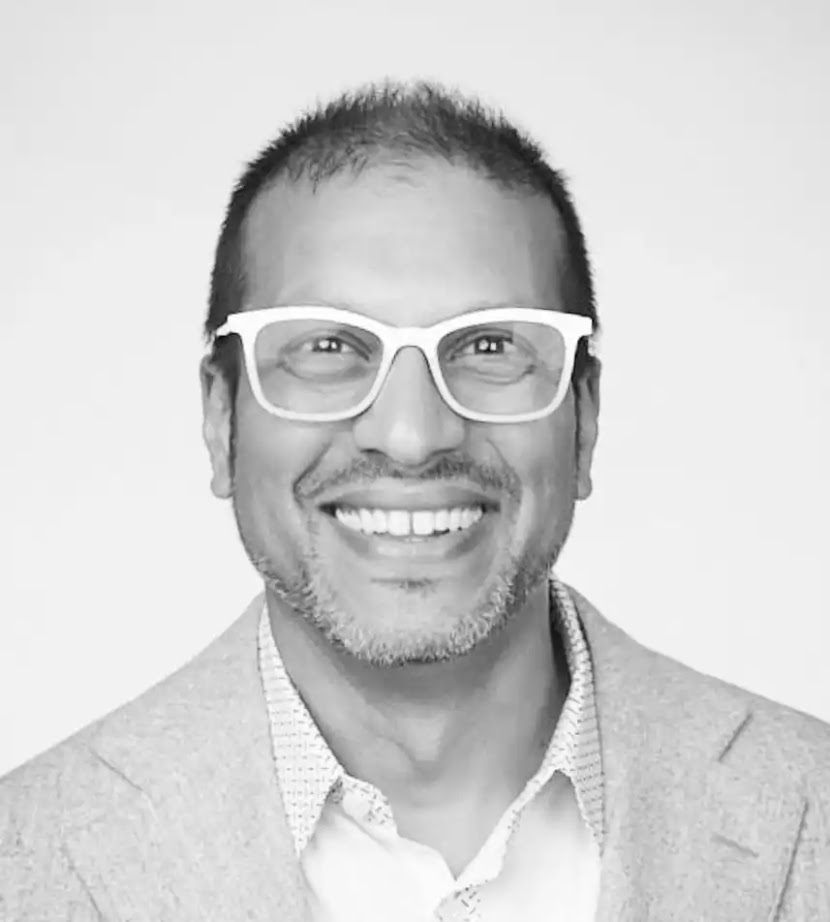
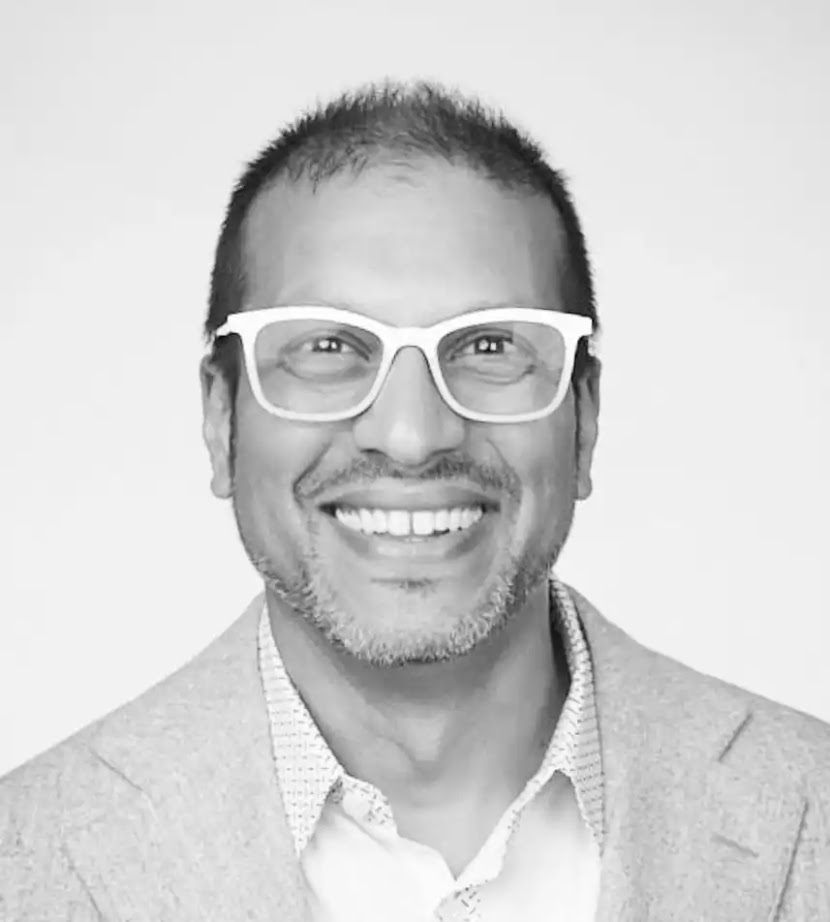
Rajiv Shah: For the past decade, my professional background has been in data science. I work at Hugging Face where I help enterprises solve their challenging problems using open source AI.
As a company, Hugging Face is devoted to building the open source AI community.
Hyperight: During the Data Innovation Summit 2024, you will share more insights on “Evaluation Techniques for Large Language Models“ – a highly relevant and important topic in the realm of LLM revolution. What can the delegates at the event expect from your presentation?
Rajiv Shah: People should walk away with ideas for building better models and having more confidence in them. As AI grows in complexity, it’s more important to ensure it’s actually solving the problem that we care about. Too often I have seen people grab the latest technology. However, a mismatch between its capabilities and end users’ needs hinders widespread usage. Evaluation is a crucial link that helps us build more useful models in less time.
During my talk, I want people to understand the role of evaluation. I’ll also cover some of the best techniques for working with generative AI/Large Language Models (LLMs).
Hyperight: What distinguishes Hugging Face’s approach to the development and deployment of generative AI from other organizations in the AI space?
Rajiv Shah: Hugging Face dedicates itself to the open-source community, focusing not on a particular model or hardware stack but on building tools and infrastructure for sustained open-source growth.
Hyperight: What was the journey like for Hugging Face in adopting machine learning technologies, especially in natural language processing? What contributed to the company’s current standing in the field?
Rajiv Shah: Hugging Face was a pioneer in bringing the latest advances, like transformers, to be easily and widely used. For the last five years, they have been a crucial tool for data scientists and developers to harness the latest advancements in AI. While Hugging Face started in natural language processing, over time, the company has expanded to all sorts of modalities of data including images and audio. Today, Hugging Face hosts over a million models and millions of users visit the site regularly.
Hyperight: What resources and tools were essential for initiating and sustaining this journey?
Rajiv Shah: It’s a community! Hugging Face has thousands of people submitting code, writing tutorials, and educating people about open source. The greatest resource is the time, as well as willingness of all sorts of people to contribute.
Hyperight: Can you elaborate on any challenges in evaluating the effectiveness and performance of large language models in enterprise settings, as well as limitations of existing methods, including their impact on optimal LLM selection?
Rajiv Shah: There is a robust market for LLMs from proprietary APIs like OpenAI to open models like Meta’s Llama model. Each of these approaches come with their tradeoffs. While an API is quick to get started with, there is also a lack of control and the need to send data outside of your environment. With an open model, you need a team and resources that can deploy a model with your own environment. I often see major corporations incorporating a mix of these approaches.
With the growth of LLMs, there are thousands of LLMs available now from providers like Hugging Face. The challenge lies in selecting the appropriate Large Language Model (LLM) for your needs. In my evaluation talk, I aim to provide some guidance for helping people through this decision.
Hyperight: Can you provide insights into the practical applications of AI that you find most promising for enterprise teams, especially in the context of LLMs?
Rajiv Shah: The biggest use cases I see are customer support applications like chatbots, code generation tools like Github Copilot, and question/answer systems built on a RAG (Retrieval-augmented generation) framework. Beyond that, people are often trying to substitute LLMs in traditional NLP use cases like text classification or text extraction.
Hyperight: From your perspective, how crucial are interpretability and explainability in the adoption of large language models, especially in industries emphasizing regulatory compliance?
Rajiv Shah: Interpretability and explainability are critical for regulatory compliance. The opaqueness of LLMs means that they will not meet regulatory requirements. However, LLMs will have a significant impact by creating data used in smaller regulated models. They will also serve as advisors in an increasing number of use cases.
Hyperight: According to you, what AI trends can we expect in the upcoming 12 months?
Rajiv Shah: Algorithms: Alternatives to traditional transformers will grow with improved performance, and enterprises will use some of those alternatives in production by the end of the year.
Generative AI Hype: We will see some of the massive spending by enterprises on Generative AI pullback as they realize the ROI is not meeting their expectations. We are already seeing companies struggling to get their models in production.
Startups: Shakeup among Generative AI startups as many fail to meet their projected revenue targets. We are already seeing startups close down or pivot.
For the newest insights in the world of data and AI, subscribe to Hyperight Premium. Stay ahead of the curve with exclusive content that will deepen your understanding of the evolving data landscape.
Add comment