Data Innovation Summit turns five next March. Along the way, we have had fantastic speakers unselfishly sharing their knowledge on stage with their peers. Without them, this journey would be impossible.
This interview is part of an interview series dedicated to humanising Data and AI innovation and celebrating speakers who have presented at the Data Innovation Summit. The emphasis lies on the Data/AI people/practitioners, their professional journey and their stories.
Apart from the hype around machine learning and deep learning, another aspect of AI has been gaining momentum – that of symbolic AI and knowledge graphs in particular. Symbolic artificial intelligence was a dominant paradigm three decades until the 1980s, but it’s made a comeback by finding practical purposes with companies – the biggest one is Google in their search engine.
Another field where knowledge graphs have found a fruitful application is in HR with matching the right people with the right job. Panos Alexopoulos brought knowledge graphs on stage at Data Innovation Summit 2018 to share his lessons from building one for the labour market. We had a chat with him about the state and progress of knowledge graphs after nearly 2 years.
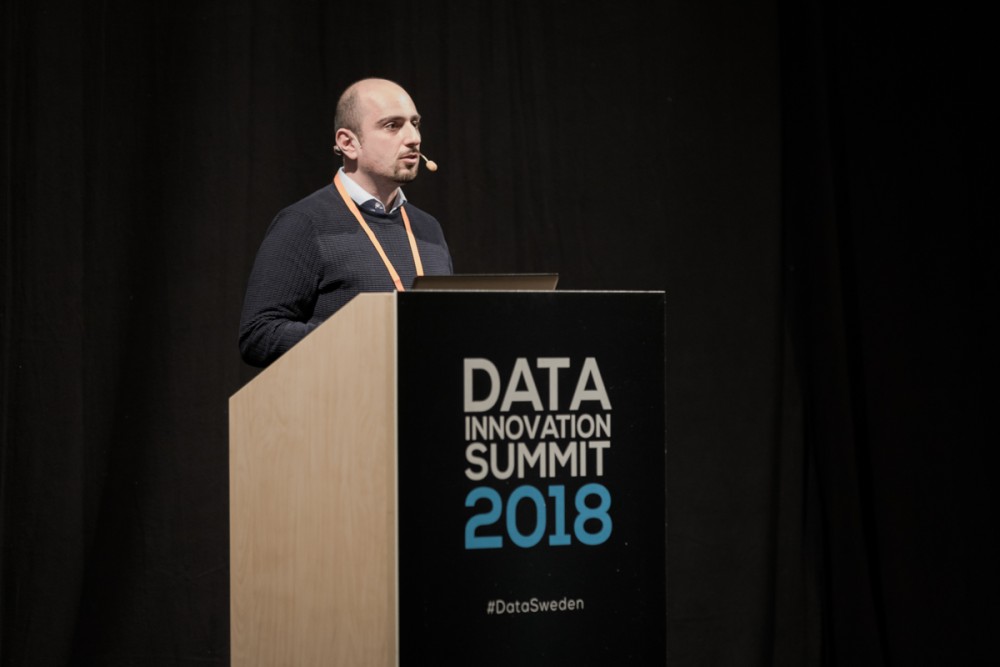
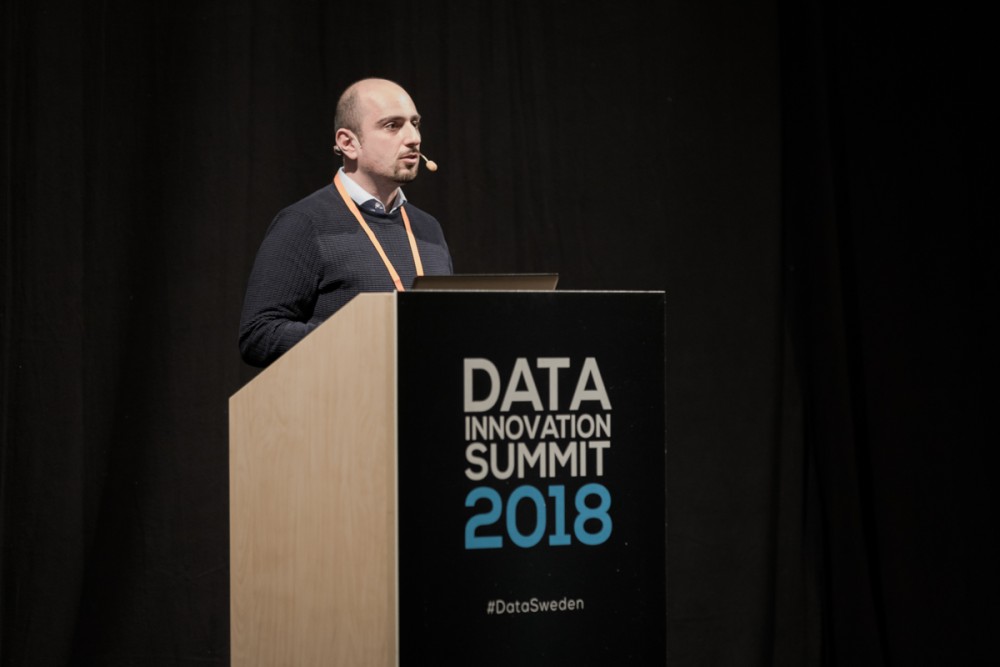
Hyperight: Hi Panos, it’s great to talk again! You were a speaker at Data Innovation Summit 2018. To refresh our memories and introduce yourself to our readers, please tell us a bit about yourself and the company you are coming from.
Panos Alexopoulos: Hi Ivana, thanks for having me back. I am coming from Amsterdam and Textkernel, a company that develops and provides software that matches people and jobs by analyzing and matching CVs and job vacancies in an intelligent way. My role there is making sure that the systems that perform these functions have all the domain knowledge they need about professions, skills and qualifications, in order to make sense of what people write and mean when they look for an employer or employee. This knowledge has the form of a knowledge graph, an increasingly popular data representation paradigm that gives emphasis on the explicitness and machine-interpretability of data meaning.
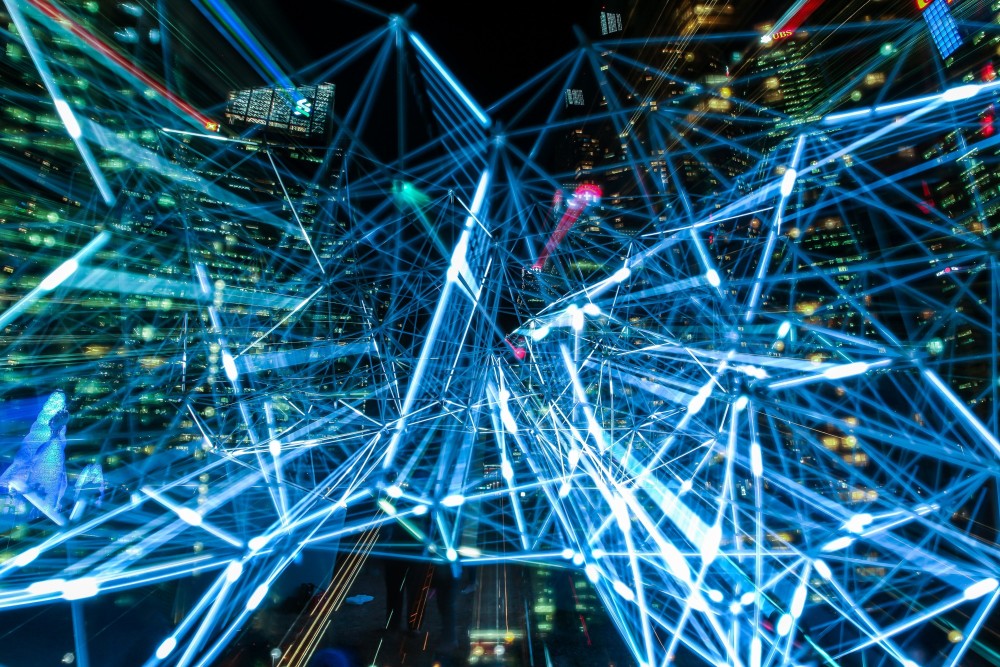
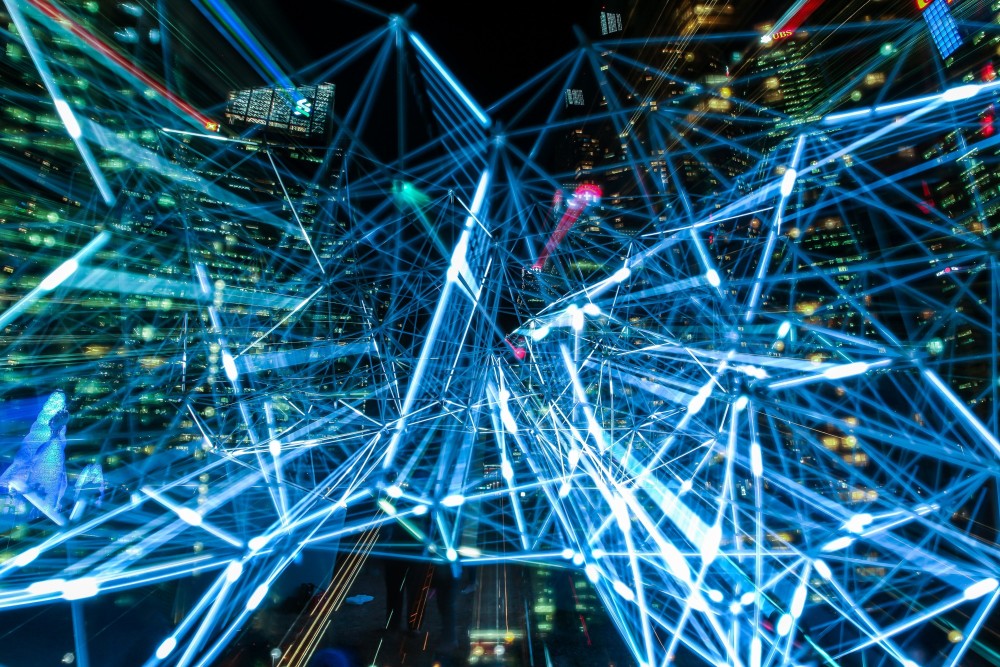
Hyperight: Next year we are celebrating our 5th anniversary. A lot has changed with data and advanced analytics during these 5 years. From your point of view, where do we see the biggest changes and advancements we have had?
Panos Alexopoulos: I don’t know how big a change it is, but knowledge graphs (and more generally symbolic AI approaches) are gaining momentum as a complement of machine learning and statistical methods. One reason is that in many areas, like for example language understanding, machine learning is still pretty far from being effective enough without the assistance of some human-provided domain knowledge. Another reason is that there is a push for machine learning algorithms and systems to become more transparent and explainable, and knowledge graphs have been shown to help towards that goal.
Hyperight: In your 2018 presentation, you discussed building, applying and evolving knowledge graphs in organizations. You are also professionally involved in developing, delivering and maintaining a large multilingual Knowledge Graph about the HR and Recruitment domain. Could you give a short recap of how knowledge graphs have revolutionised search and matching in recruitment in the past several years?
Panos Alexopoulos: I wouldn’t use the term “revolutionize” but rather “significantly improved”. A prerequisite for a good automatic match between a job vacancy and a candidate’s profile is to enable the machine to go beyond keywords and start considering concepts and relations. For that, the machine needs to be able to map keywords to concepts (such as professions and skills) and know when two keywords have the same or similar meaning. Developing and combining this knowledge with sophisticated machine learning algorithms, has enabled Textkernel’s products to be leaders in the recruitment technology field.


Hyperight: What about the challenges with development, exploitation and evolution of knowledge graph? Have you managed to surpass them, or are you dealing with even more complex challenges nearly 2 years after?
Panos Alexopoulos: We are becoming better in all these aspects but of course some core challenges remain. By “core” I mean challenges caused by the variety, ambiguity, implicitness, vagueness and other characteristics of human language, thinking and communication. Despite the advances in AI, we are still pretty far from tackling all of them in a complete way. But that doesn’t mean we should stop trying.
Knowledge graphs and more generally symbolic AI approaches are gaining momentum as a complement of machine learning and statistical methods.
Hyperight: Talking about the decade to come, what are your future outlooks for the development and use of knowledge graphs for 2030?
Panos Alexopoulos: I don’t know if the term “knowledge graph” will still be used in 2030 or some new term will take its place but I believe that symbolic AI methods will still be in use, hopefully in harmonic and seamless collaboration with Machine Learning and other AI paradigms.
Add comment