At the Data Engineering and DataOps Stage at the eighth edition of the Data Innovation Summit, there will be one exciting presentation by the representatives of Twitter: Rishabh Misra and Jigyasa Grover, both Senior Data Engineers. During their session, they will talk about data curation – a very rudimental aspect of machine learning, as they say in this interview.
The interview discovers what skills are needed for this approach that Twitter used for data curation and dataset construction. Besides the skills, they advise having the technical knowledge to identify and curate meaningful and unbiased datasets.
We hope that you find other interesting insights that will motivate you to enroll for their session at the most influential data, AI and advanced analytics event in the Nordics.
Hyperight: Can you tell us more about yourself and your organization? What are your professional background and current working focus?
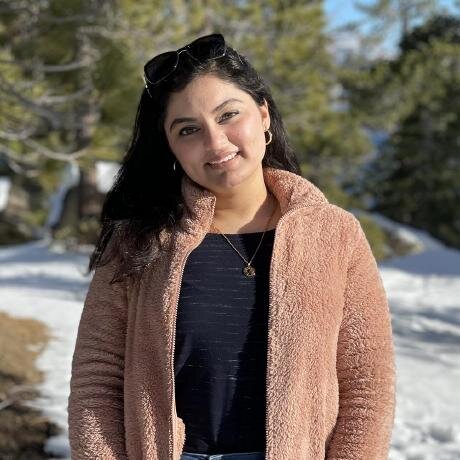
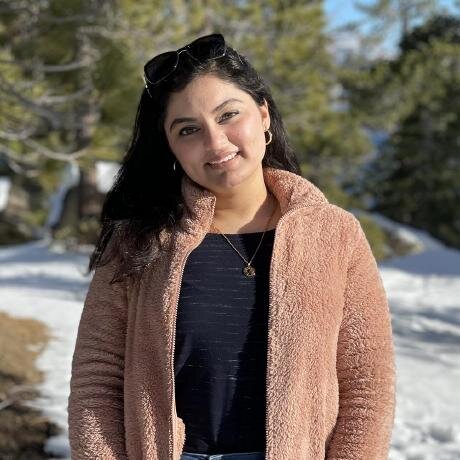
Jigyasa Grover: I am currently working as a Senior Machine Learning Engineer at Twitter, where I have been working in the Online Ads Prediction & Ranking domain for 4 years now. I am spearheading a variety of ML projects that are directed toward increasing the revenue for the company while enhancing the advertiser and user experience on the platform.
Having graduated from the University of California, San Diego, with a Masterās degree in Computer Science with an Artificial Intelligence specialization, my journey is also highlighted by a myriad of experiences from my brief stints at Facebook, the National Research Council of Canada, and the Institute of Research & Development France involving data science, mathematical modeling, and software engineering. I have co-authored a book on Data-Centric ML titled āSculpting Data for MLā and my latest research āDo not fake it till you make it!ā, which is a synopsis of trending fake news detection methodologies on social media using deep learning got published in a world-renowned Springer book series. Having the honor of 10 international awards, I am an avid proponent of open-source and credit the access to opportunities and my career growth to this sphere of community development.
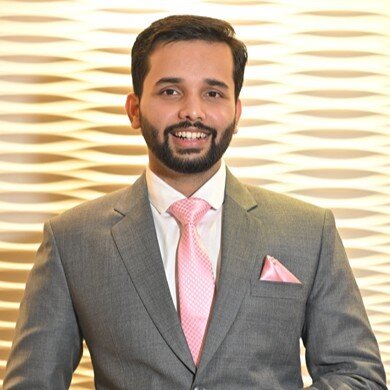
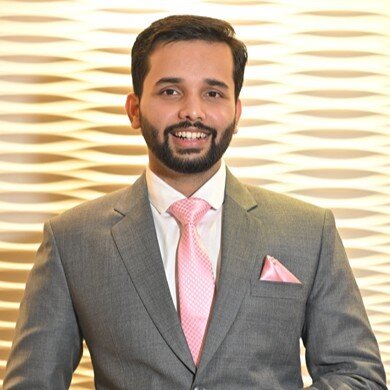
Rishabh Misra: I am a Senior Machine Learning Engineer at Twitter and co-author of the book “Sculpting Data for ML”. I work on surfacing relevant content on Twitter across home feed and replies using recommendations and ranking techniques.
Previously, I have worked at Amazon after getting my Masterās degree from UC San Diego in Artificial Intelligence. I have worked extensively with problems relating to user behavior modeling and have published in some top Machine Learning conferences like RecSys, ACL, and WSDM. Holistically, I love to combine my engineering experiences in designing large-scale systems with applied Machine Learning experiences to develop distributed Machine Learning relevance systems for improving end-user Experience.
Hyperight: During the Data Innovation Summit 2023, you will share more on “Sculpting Data for Machine Learning”. What can the delegates at the event expect from your presentation?
Jigyasa & Rishabh: There is a modern bloom of social networks, online shopping portals, blogs, video streaming platforms, and so many other knowledge and experience sharing platforms enabled with all kinds of media, be it textual, visual, or audio. Consequently, a vast magnitude of raw data is available via numerous sources nowadays. This talk aims to provide an in-depth guide about one of the most rudimentary aspects of Machine Learning – Dataset Curation. It will walk scientists, engineers, researchers, investors, policymakers, and business leaders through the journey of dataset curation with real-world examples based on our experiences. The goal is to institute the significance of data, especially in its worthy format, and recognize its effect on advancing Machine Learning systems’ capability.
Hyperight: What was the need for any organization to think of data curation and dataset construction processes like the ones you will address in your presentation?
Jigyasa & Rishabh: Globally business-oriented corporations are increasingly investing in Machine Learning as they realize the value technology adds to their product and business. Industry prioritizes profit-generating logic and high scale performance higher than novelty and advancement of theoretical knowledge. Therefore, most use cases involve utilizing learning algorithmsā performance on a large scale to improve the user experience or revenue generation. Established organizations seldom have obstacles in obtaining computation power, hiring folks with expertise in corresponding domains, or accessing relevant data. The challenge, however, comes in scaling up their solutions to their massive user base. Any organization would have a lot of unstructured data available on its hands as raw logs; however, developing an efficient data processing pipeline remains a task. For creating such resilient pipelines, apart from the relevant technological knowledge, we also require the skills to identify and curate meaningful and unbiased datasets from a sea of unstructured data.
Hyperight: Can you tell us more about the journey of implementing such processes and steps for data curation and dataset construction so far? What was the starting point, where are you now, and what is next?
Jigyasa & Rishabh: Oftentimes, depending on why we want to collect data, there are different ways to approach building good quality datasets. However, in a broader sense the different search techniques boil down to these key processes: formal problem definition, essential data signal determination, metadata association, data volume requirement, and data integration from multiple sources.
Hyperight: What resources and tools did you need to start and continue this journey?
Jigyasa & Rishabh: Being ML engineers in the industry, we feel having a problem-solving mindset is important topped with fundamental skills like software engineering, system design, and data modeling. As we grow in our career, we are realizing that domain knowledge plays a pivotal role in thinking through the problem and coming up with effective solutions. Another useful skill is the art of storytelling which involves Communicating oneās approaches by articulating the problem, end-to-end solution backed with data-driven analysis, and providing actionable insights.
Hyperight: Did you face any challenges throughout data curation and dataset construction, and what were the ways to overcome them?
Jigyasa & Rishabh: Once we have narrowed down the source to use for dataset curation and acquired the knowledge of the tools we need, it is very tempting to start scraping the content right away. However, It is crucial to be cognizant of the selected sourceās āTerms & Conditionsā before starting the extraction process. In the era where data protection and privacy regulations are tightening, we should be very mindful of what data we can use, when and how.
Hyperight: Do you have any recommendations for anyone interested in starting a similar journey based on the lessons learned from yours?
Jigyasa & Rishabh: One of top pieces of advice will be to hone your technical know-how and find yourself opportunities to work in multiple realms for you to gain enough experience be it in the form of internships, volunteering, curriculum, and so on. These varied exposures will help you decide on your domain of expertise. For students particularly interested in Data Curation and Responsible AI, like budding data scientists, ML engineers, etc. put in the effort to read up on the principles and devise ways how you can implement them in practical life. Our belief is that ideas are nothing without implementation, so make sure you hone those execution skills and build your portfolio accordingly!
Hyperight: According to you, what AI trends can we expect in the upcoming 12 months?
Jigyasa & Rishabh: Technology is touching every aspect of our life and in a way shaping humankind in the present era. With its significance augmenting each passing day, it is highly crucial to have certain checks in place to promote its ethical growth and avoid socio-economic damage to society. Especially with modern-day AI systems, even a slight crack in their design can lead to disastrous outcomes propelled by cyber-attacks, reverse engineering, and leakage of sensitive data like personal conversations, financial transactions, medical history, and so on. Therefore, it is imperative to retain the confidentiality of data, maintain the privacy of proprietary design, and stay compliant with the latest regulations and policies.
Add comment